Unveiling the immunomodulatory mechanisms of pineapple metabolites: A multi-modal computational analysis using network pharmacology, molecular docking, and molecular dynamics simulation
Abstract
The exploration of the immunomodulatory potential of pineapple metabolites holds promise for substantial implications across several fields, encompassing medicine, pharmacology, nutrition, and public health. This study explores potential immune-regulating properties of secondary pineapple metabolites beyond bromelain, using computational techniques. Pineapple juice's secondary metabolites were identified via LC-MS-based metabolomics, selected using KNApSAcK Kanaya and Dr. Duke's databases. A heatmap was generated with Orange v.3.27.0. Bioactivity predictions utilized the PASS Online webserver, while ADMET properties were forecasted. Network pharmacology explored metabolite interactions with protein targets. Molecular docking focused on compounds against receptors, choosing robust interactors for dynamic simulations. Our findings revealed that certain metabolites from pineapple juice/fermented juice exhibited interactions with proteins associated with pro-inflammatory cytokines. Specifically, the molecular docking results indicated that the carbohydrate moiety of bromelain (CMB) interacted strongly with TLR2, while 9,10-Dihydroxystearic acid showed strong interactions with NLRP3 (inflammasome). The flexibility and stability of these complexes were further assessed through molecular dynamics simulations conducted over a 50-ns time period. The MM-PBSA calculations also indicated low binding free energies between these complexes, suggesting strong molecular interactions. These findings suggest that CMB may interact with TLR2 and 9,10-Dihydroxystearic acid may interact with NLRP3, highlighting their potential as immunomodulatory agents. However, further experimental studies are warranted to confirm the therapeutic efficacy of these molecules and investigate their mechanisms of action in vivo.
INTRODUCTION
The immune system serves as the body's primary defense mechanism against external invaders like bacteria, viruses, and other pathogens, and also has a role in eliminating damaged or abnormal cells within the body. It operates on two primary levels: innate and adaptive immunity [1]. The innate immune system functions as a rapid early warning system, providing immediate but generalized protection, while the adaptive immune system offers a more targeted and specialized response to pathogens [2]. Both layers work in concert to maintain homeostasis and ensure optimal physiological functioning [3,4]. This equilibrium is critical for optimal physiological functioning and overall well-being. However, the immune system is a double-edged sword. While its proper functioning is essential for health, dysregulation or imbalance in immune responses can have detrimental effects [5].
When the immune system is overactive, it can start attacking healthy tissues and cells, leading to autoimmune diseases like rheumatoid arthritis, lupus, and multiple sclerosis [6]. These conditions often require life-long management and can significantly impair the quality of life of affected individuals. Conversely, an underactive immune system can result in inadequate defense against pathogens, leading to frequent infections and increased susceptibility to diseases [1,7]. Additionally, imbalances in the immune system can trigger excessive inflammatory responses, a hallmark of numerous health issues ranging from acute conditions like sepsis to chronic diseases like obesity, cardiovascular disease, and diabetes [8,9]. This exaggerated inflammation, often described as a "cytokine storm" when particularly severe, can lead to widespread tissue damage and organ failure if not adequately managed [10,11].
Moreover, dysregulation of immune responses can have more subtle, yet pervasive, effects on health. For instance, chronic low-level inflammation is increasingly being recognized as a contributing factor to aging and age-related diseases, including cancer and neurodegenerative conditions like Alzheimer's disease [12,13]. Therefore, modulating immune system activity is not only crucial for fighting diseases but also for preventive health measures aimed at maintaining long-term well-being. Given the complex nature of the immune system and its central role in health and disease, there is a pressing need for therapeutic agents that can modulate immune activity in a targeted manner.
In recent years, scholarly interest has intensified in the exploration of natural compounds possessing immunomodulatory properties, given their potential to serve as safer and more accessible therapeutic interventions. Pineapple (Ananas comosus (L.) Merr.), a fruit celebrated for its nutritional merits, is not only consumed widely but also recognized as a medicinal plant in various communities [14]. While its enzyme complex, bromelain, has garnered significant attention for its anti-inflammatory and immunomodulatory prowess, the fruit's secondary metabolites, which exhibit a spectrum of bioactive properties including immunomodulation, anti-inflammatory, and antithrombotic effects, remain relatively underexplored [15]. Immunomodulators are chemical compounds that can modulate the immune system, either by enhancing (immunostimulants) or suppressing (immunosuppressants) the production of serum antibodies [16]. Several fruits, whether in raw or fermented forms, contain secondary metabolite compounds with immunomodulatory activity [17].
The objective of this study was to examine the secondary metabolite profile of both raw and fermented pineapple juice in an effort to elucidate the immunomodulatory mechanisms of these pineapple metabolites. To achieve this goal, a multifaceted approach was utilized, integrating computational methods such as network pharmacology, molecular docking, and molecular dynamics simulation, in order to rigorously analyze and comprehend the complex interaction between pineapple metabolites and the immune system. The findings of this study are anticipated to contribute to the advancement of natural product research and immunology. A schematic overview of the strategies employed to uncover these immunomodulatory mechanisms is presented in Figure 1.
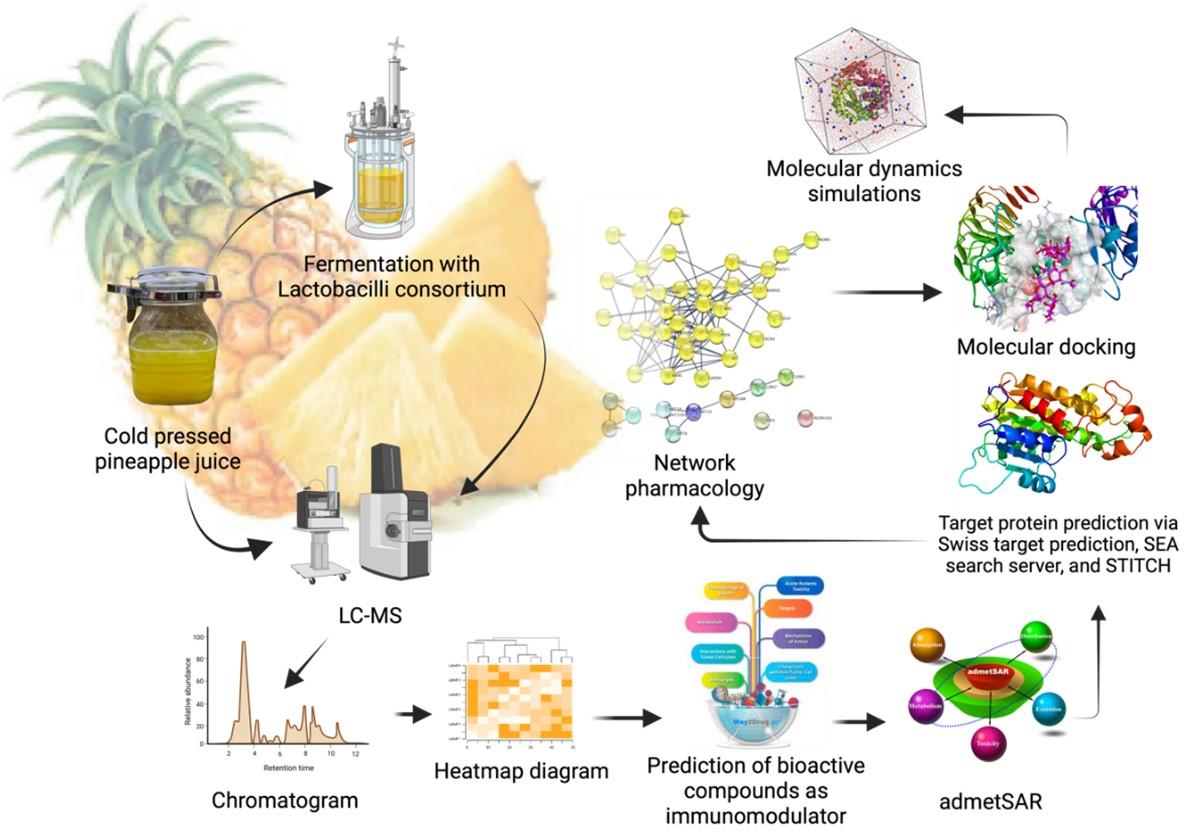
MATERIALS AND METHODS
Sample preparation and fermentation
The Lobong pineapple variety was obtained from the village of Lobong, Bolaang Mongondow District, located in North Sulawesi, Indonesia (0° 45' 56" North, 124° 15' 41" East). The cold press juice method was utilized to extract pineapple juice from the collected samples. This method preserves the activity of enzymes and the integrity of metabolites by avoiding the use of heat. Subsequently, a portion of the pineapple juice was subjected to fermentation using a consortium of Lactobacillus bacteria for three days at room temperature.
Liquid chromatography-mass spectrometry (LC-MS) analysis
Metabolite analysis of pineapple juice and fermented pineapple juice was conducted using liquid chromatography-mass spectrometry (LC-MS). In this study, a Dionex UltiMate 3000 RSLCnano System was coupled with a Q Exactive HF-X (Thermo Fisher Scientific) and screened with electrospray ionization (ESI) in both positive (ESI+) and negative (ESI-) ion modes. The LC system employed an ACQUITY UPLC HSS T3 column (100×2.1 mm, 1.8 μm) with Ultimate 3000LC. The mobile phase consisted of solvent A (0.05% formic acid in water) and solvent B (acetonitrile) with a gradient elution profile as follows: 0-1.0 min, 95% A; 1.0-12.0 min, 95-5% A; 12.0-13.5 min, 5% A; 13.5-13.6 min, 5-95% A; 13.6-16.0 min, 95% A. The flow rate of the mobile phase was set at 0.3 mL/min. The column temperature was maintained at 40°C, while the sample manager temperature was set at 4°C. The mass spectrometry parameters for ESI+ and ESI- modes were as follows:
ESI+: heater temperature of 300°C; sheath gas flow rate, 45arb; aux gas flow rate, 15arb; sweep gas flow rate, 1arb; spray voltage, 3.0KV; capillary temperature, 350°C; S-lens RF level, 30%.
ESI-: heater temperature of 300 °C, sheath gas flow rate, 45arb; aux gas flow rate, 15arb; sweep gas flow rate, 1arb; spray voltage, 3.2KV; capillary temperature, 350°C; S-lens RF level, 60%.
Selection of bioactive compounds from regular and fermented pineapple juice
The LC-MS data was scrutinized manually in order to distinguish secondary metabolites from primary metabolites. To assess the content of secondary metabolites, a comparison was made between the LC-MS data and a list of bioactive compounds obtained from the KNApSAcK Kanaya database and Dr. Duke's Phytochemical and Ethnobotanical database. The aim was to generate a consolidated list of bioactive compounds that were identified in both databases and through LC-MS analysis, as a secondary step in the selection of bioactive compounds.
Generation of heat-map diagrams
The bioactive compounds selected from both the LC-MS data list and the database, as well as the bioactive compounds derived from basic compounds in the database, were combined using Microsoft Excel (Windows Office 2010). Subsequently, the list of bioactive compounds along with their corresponding relative abundance values was visualized using the Orange v.3.27.0 software (Ljubljana, Slovenia) by creating a heat-map diagram. The heat map was generated without clustering, and diverging protanopic (for bioactive compounds found in both the LC-MS data list of both ions) and diverging tritanopic (for the compilation result with derivative compounds) color palettes were employed.
Prediction of bioactive compound activity as immunomodulators
For each compound identified in the second stage of selection, its canonical structure and isomeric SMILE (simplified molecular-input line-entry system) were searched in the PubChem database (https://pubchem.ncbi.nlm.nih.gov/). Subsequently, the secondary metabolites from pineapple juice and fermented pineapple juice were subjected to WAY2DRUG PASS prediction to assess their potential as immunomodulators. The Pa (probability to be active) values of the compounds were utilized in the data mining process, as they provide accurate information on the potential of a compound as an immunomodulator.
Prediction of the AdmetSAR of the bioactive compounds
In this study, the ADMET (absorption, distribution, metabolism, excretion, and toxicity) structure-activity relationship (SAR) of bioactive compounds was analyzed. To achieve this, canonical SMILE (simplified molecular input line entry system) structures for each selected bioactive compound were obtained from the PubChem database (https://pubchem.ncbi.nlm.nih.gov/). These SMILE structures were then used as keywords for data search in the LMMD AdmetSAR database version 2.0 (http://lmmd.ecust.edu.cn/admetsar2) and Protox version II (https://tox-new.charite.de/protox_II/index.php?site=compound_input) to retrieve ADMET-related information.
Target protein prediction
The predictions for target proteins were obtained from three different databases: Swiss Target Prediction (http://www.swisstargetprediction.ch), SEA Search Server (https://sea.bkslab.org/), and STITCH (http://stitch.embl.de/). In the case of Swiss Target Prediction and SEA Search Server, each compound’s target protein was predicted individually using its SMILE structure as the search keyword. Target proteins for each bioactive compound were then chosen based on a probability value from SWISSTarget of greater than 50% (>0.5) and TC or Tanimoto similarity (SEA). On the other hand, STITCH allowed for the input of a list of bioactive compound names and keywords via its multiple name menu. Additional settings included selecting Homo sapiens as the organism type and establishing a minimum required interaction score of 0.700.
Network pharmacology analysis
The compilation of target proteins from the three databases was inputted into the multiple protein menu of the STRING database (https://string-db.org/). Additional settings were applied, including selecting Homo sapiens as the organism type, changing the meaning of network edges to confidence, and setting the minimum required interaction score to 0.700. Once the network was updated based on these settings, the visualization results were downloaded in tab-separated values (tsv) format from the table/export menu. The downloaded .tsv file was then imported into Cytoscape v.3.8.2 software for network analysis using the tools menu, followed by biological process prediction involving target proteins using BINGO in the GOlorize app menu.
Molecular docking
The molecular docking study was conducted using Maestro 12.8 of the Schrödinger software 2021.2 version, wherein the selected compounds were docked against the selected receptors. Protein preparation was performed using the 'Protein Preparation Wizard' module, where the target proteins (TLR2 and NLRP3) were prepared by adding hydrogen atoms, creating disulfide bonds, and removing water and other heteroatoms. The TLR2 protein target was chosen based on the results of network pharmacology analysis, while NLRP3 was chosen due to the consideration that this protein is related to inflammation. Toll-like receptor 2 (TLR2) is suggested to initiate the activation of NLRP3 inflammasome [18]. The NLRP3 inflammasome is a multi-molecular complex that acts as a molecular platform to mediate caspase-1 activation, leading to IL-1β/IL-18 maturation and release in cells stimulated by various pathogen-associated molecular patterns (PAMPs) or damage-associated molecular patterns (DAMPs). This inflammasome plays an important role in the innate immunity as its activation can further promote inflammation [19].
H-bond assignments for protein optimization were carried out based on sample water orientations using PROPKA pH 7.0. The protein minimization was performed with converging heavy atoms to an RMSD of 0.3 Å using the OPLS419 force field. The ligand structures were prepared in 3D and minimized using the OPLS4 force field at pH 7±2 with the 'LigPrep' module. The active site of target proteins was determined using the ‘SiteMap’ module, and a 20*20*20 Å3 area was created by the ‘Receptor Grid Generation’ module for molecular docking. The docking was carried out using the ‘Glide XP’ module. Protein-ligand interactions in 2D and binding mode analysis in 3D were performed using Discovery Studio Visualizer v.2021.
Molecular dynamics simulation
Molecular dynamics simulation was performed with Gromacs (GROningen MAchine for Chemical Simulations) 2019.2 version to investigate the stability of the complexes (TLR2-44263865 and NLRP3-89377). The ligands (44263865 and 89377) were chosen based on their optimal interaction with each target protein. The topology of the ligands 44263865 and 89377 was created using the GlycoBioChem PRODRG2 server. The topology file of TLR2 and NLRP3 was generated using the GROMOS 43a1 force field and the SCP water model. The energy of the protein-ligand-ion-solvent system was minimized using the steepest descent integrator algorithm with 5000 steps. The system was equilibrated with 0.3 ns NVT (constant Number of particles, Volume, and Temperature) and 0.3 ns NPT (constant Number of particles, Pressure, and Temperature) stages at 1 atm pressure and 300 K temperature, using the V-rescale thermostat and the Parrinello-Rahman barostat. The 50 ns molecular dynamics simulation was performed with a leap-frog MD integrator. A 50 ns molecular dynamics simulation was performed using a leap-frog MD integrator. Trajectory analysis was conducted using gmx scripts, including measurements such as root-mean-square deviation (RMSD), root-mean-square fluctuation (RMSF), radius of gyration (Rg), and solvent accessible surface area (SASA). The MD trajectory analysis results were visualized using VMD - Visual Molecular Dynamics, Discovery Studio Visualizer, and graphs were generated using GraphPad Prism.
Calculation of the MM-PBSA binding-free energy
The molecular mechanics/Poisson–Boltzmann surface area (MM/PBSA) approach was employed to estimate the free energy of binding between the selected compounds and the selected receptor rs. The g_mmpbsa script was utilized to compute the binding-free energy (BFE), taking into account the contributions from Van der Waals interactions, polar solvation, and electrostatics. The non-polar energy contribution was calculated based on the solvent accessible surface area (SASA). The MMPBSA free binding energy was calculated using the following equation: ΔGbind=GComplex−Gprotein−Gligand
RESULTS
Selection of secondary metabolites as bioactive compounds and the creation of heat-map diagrams
The bioactive compounds acquired from Kanaya's KNApSAcK database (Supplementary Table 1) and Dr. Duke's Phytochemical and Ethnobotanical databases (Supplementary Table 2) were employed to select bioactive compounds from liquid chromatography-mass spectrometry (LC-MS) data. Subsequent to the selection phase, a total of 12 bioactive compounds were identified as pineapple metabolites with prospective immunomodulatory capabilities. These compounds were concurrently present in both the dataset derived from data mining techniques and the LC-MS analysis, indicating their significant potential in the relevant field. These compounds include 1-O-caffeoyl-3-O-feruloylglycerol, ascorbic acid, caffeic acid, citric acid, ferulic acid, linoleic acid, oleic acid, palmitic acid, palmitoleic acid, pantothenic acid, serotonin, and stearic acid (Figure 2).
Beyond the primary 12 compounds of interest, an additional 21 derivative compounds were discerned within the heatmap analysis (Figure 3). Concurrently, an array of compounds including caffeic acid, citric acid, ferulic acid, pantothenic acid, and (-)-threo-isodihomocitric acid were detected in both ion forms during the liquid chromatography-mass spectrometry (LC-MS) analysis. Based on the relative abundance data procured from the LC-MS analysis, it is observed that nearly all compounds are present in both sample types. However, 1-O-caffeoyl-3-O-feruloylglycerol and ethyl vanillin isobutyrate were found to be significantly abundant exclusively in F-JN sample.
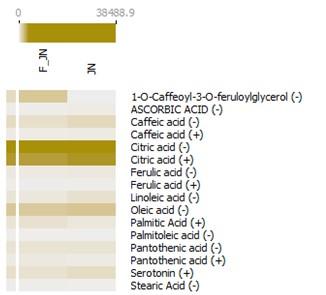
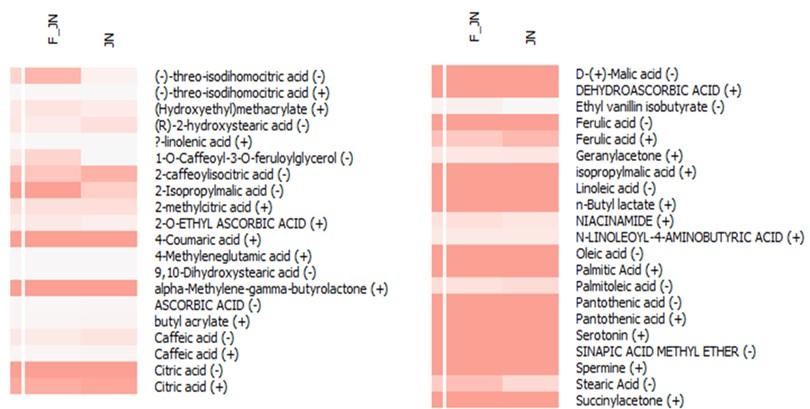
Prediction of the immunomodulatory potential of bioactive compounds
The assessment of the prospective bioactivity of pineapple metabolites in an immunomodulatory context was executed on a set of 34 preeminent compounds. Additionally, one compound—the carbohydrate moiety of bromelain (CMB)— which was identified solely through database-driven data mining and not via liquid chromatography-mass spectrometry (LC-MS) was also included in the analysis (Figure 4 and Supplementary Table 3). Possessing a Pa value of 0.952, CMB demonstrates high bioactivity as an immunostimulant. Moreover, CMB exhibits moderate immunomodulatory and immunosuppressive activity. 9,10-dihydroxystearic acid (9,10-dihydroxyoctadecanoic acid) possesses a Pa value of 0.514 as an immunomodulator, 0.616 as an immunostimulant, and 0.524 as an immunosuppressant. This compound is a hydroxy-fatty acid formed by hydroxy substitution at positions 9 and 10 of octadecanoic (stearic) acid. N-linoleoyl-4-aminobutyric acid (GABA linoleamide or N-linoleoyl GABA) and succinylacetone (4,6-dioxoheptanoic acid) appear to exhibit a proclivity for acting as immunostimulants, with Pa values exceeding 0.6.
Besides immunomodulatory activity, pineapple secondary metabolites were explored for their potential in anti-inflammatory, free radical scavenger, multiple interleukin antagonist, cytokine modulator, cytokine release inhibitor, prostaglandin-E2 9-reductase inhibitor, and cyclooxygenase (COX) inhibitor activities, as illustrated in Supplementary Tables 4 and 5. Supplementary Table 4 indicates that pineapple encompasses a considerable number of secondary metabolites possessing substantial prostaglandin-reductase and COX inhibitor activities.
Although not all compounds in Supplementary Table 5 exhibit high activity as anti-inflammatory agents and free radical scavengers, several compounds display moderate activity as interleukin-2 (IL-2) agonists. The carbohydrate moiety of bromelain (CMB), 1-O-Caffeoyl-3-O-feruloylglycerol, and ferulic acid all demonstrated notable free radical scavenging activity. Ascorbic acid, linoleic acid, alpha-linolenic acid, alpha-methylene-gamma-butyrolactone, and geranylacetone all possess significant anti-inflammatory properties.
Prediction of AdmetSAR properties for pineapple bioactive compounds
AdmetSAR is capable of predicting approximately 50 ADMET endpoints using the QSAR model (Supplementary Table 6). Concurrently, the ProTox II database was utilized to generate predictions for Lipinski's rule, although it only produced predictions for the compounds' toxicity (Supplementary Table 7). Individual compounds with potential toxicity and/or violations of Lipinski's rule are highlighted in bold. Lipinski's Rule of 5 (Ro5) assists in differentiating drug-like from non-drug-like molecules. Furthermore, Lipinski's rule forecasts the probable success or failure of a compound's metabolism based on its resemblance to a drug molecule, wherein at least three of the following criteria apply: molecular mass less than 500 Daltons, high lipophilicity (logP less than 5), fewer than five hydrogen bond donors, fewer than ten hydrogen bond acceptors, and a molar refractivity between 40 and 130 [20,21].
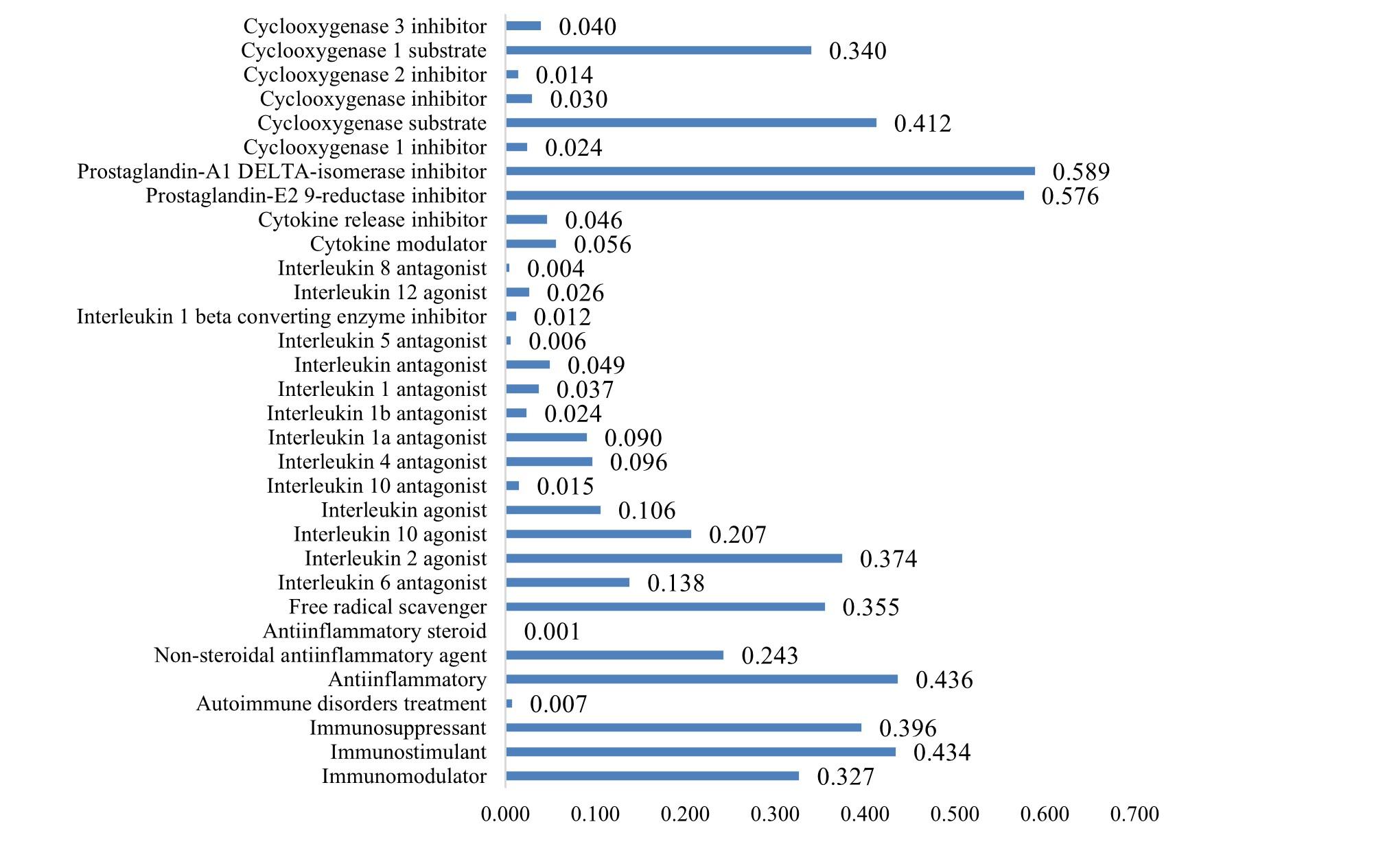
Network pharmacology analysis
Identifying compound-target protein interactions is crucial for understanding a compound's mechanism of action. The potential targets of the compounds can be discerned through network analysis. The target proteins derived from the three databases (SwissTarget, SEA Server, and STITCH) include TNFα, INF-γ, IL 6, IL 1β, ABCG2, ACAT, ACHE, ACLY, AKR1B1, AKR1B10, AKR1C3, AKR1C4, ALOX12, ALOX15, ALOX5, ALPI, AMD1, APHA, APP, BACE1, BCHE, BLA(TEM-2), CA1, CA12, CA14, CA15, CA2, CA3, CA4, CA5A, CA5B, CA6, CA7, CA9, CACNA1C, CAMK2A, CASP2, CD69, CDC25A, CDC25B, CDC25C, CES1, CES2, CNR1, CNR2, CPT1A, CPT2, CQSS, CXCL12, CYP4A4, CYSLTR1, DBH, DHPS, DNM1, DPP4, DYRK1A, EGFR, EP300, EPHX2, ESR1, ESR2, EST1, F3, FAAH, FABI, FABP1, FABP2, FABP3, FABP4, FABP5, FFAR1, FFAR4, FOLH1, FOS, G6PD-6PGL, GLO1, GPR18, GPR84, GRIK1, GRIK2, HCAR2, HDAC1, HDAC11, HDAC2, HDAC8, HDAC9, HMGCR, HSD11B1, HSD17B3, HSF1, IAP, IKBKG, JHE, JUN, KCNK2, KDM2A, KDM5A, KDM5C, LEF, LGALS3, LGALS4, LGALS8, LOX1.1, LPAR1, LPAR2, LTB4R, MAOA, MAOB, MAPT, ME2, MET, MIF, MMP1, MMP2, MMP9, MYOC, NFE2L2, NFKB1, NQO2, ODC1, OXER1, PAM, PAOX, PHF8, PLA2G2A, PLA2G2C, POL, PPARA, PPARD, PPARG, PPO2, PRP19, PTGER2, PTGER4, PTGES, PTGFR, PTGS1, PTGS2, PTPN1, RELA, SAT1, SCD, SHBG, SKA, SLC22A6, SLC22A8, SLC23A2, SMS, SNCA, SOAT1, SPHK1, SSSIM, TERT, TLR2, TLR4, TLR9, TOP1, TOP2A, TRAPPC3, TRPA1, TRPV1, TRPV2, TTR, and XDH.
The BINGO analysis from GOlorize yielded insights into the function of each compound in biological processes and the relationships between target proteins, as depicted in Figure 5. Moreover, Table 1 presents the target proteins involved in reactive oxygen species, immune response, inflammatory response, and oxidative stress, where the variables include stress, degree, betweenness centrality, and closeness centrality. The most promising target proteins are listed in Supplementary Table 8 in ascending order based on their potential.
Table 1. Target proteins generated from network analysis using Cytoscape.
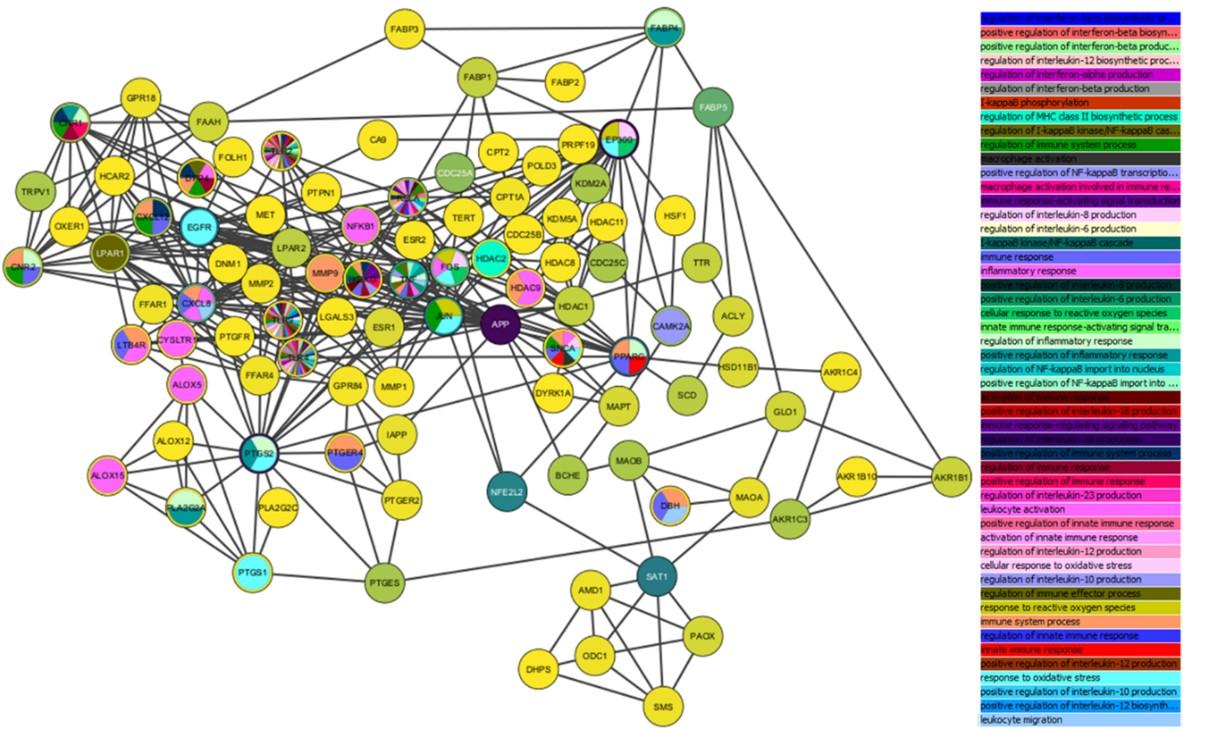
Molecular docking analysis
The selection of several proteins/receptors for further molecular docking analysis was based on the analysis of protein targets from pineapple juice/fermented metabolites. The most favorable binding modes were identified by scoring a range of configurations generated by docking analysis. The selected pineapple metabolites displayed varying docking results to the receptors NF-kB, TLR2, NLRP3, and TNF-α (Table 2). Notably, the most favorable interactions were observed between CMB and TLR2, as well as between 9,10-dihydroxystearic acid (9,10-DHSA) and NLRP3, as indicated in bold. The binding modes and schematic 2D interactions are presented in Figure 6.
Table 2. Docking results of bioactive molecules of pineapple’s metabolites on selected immunomodulatory receptors.
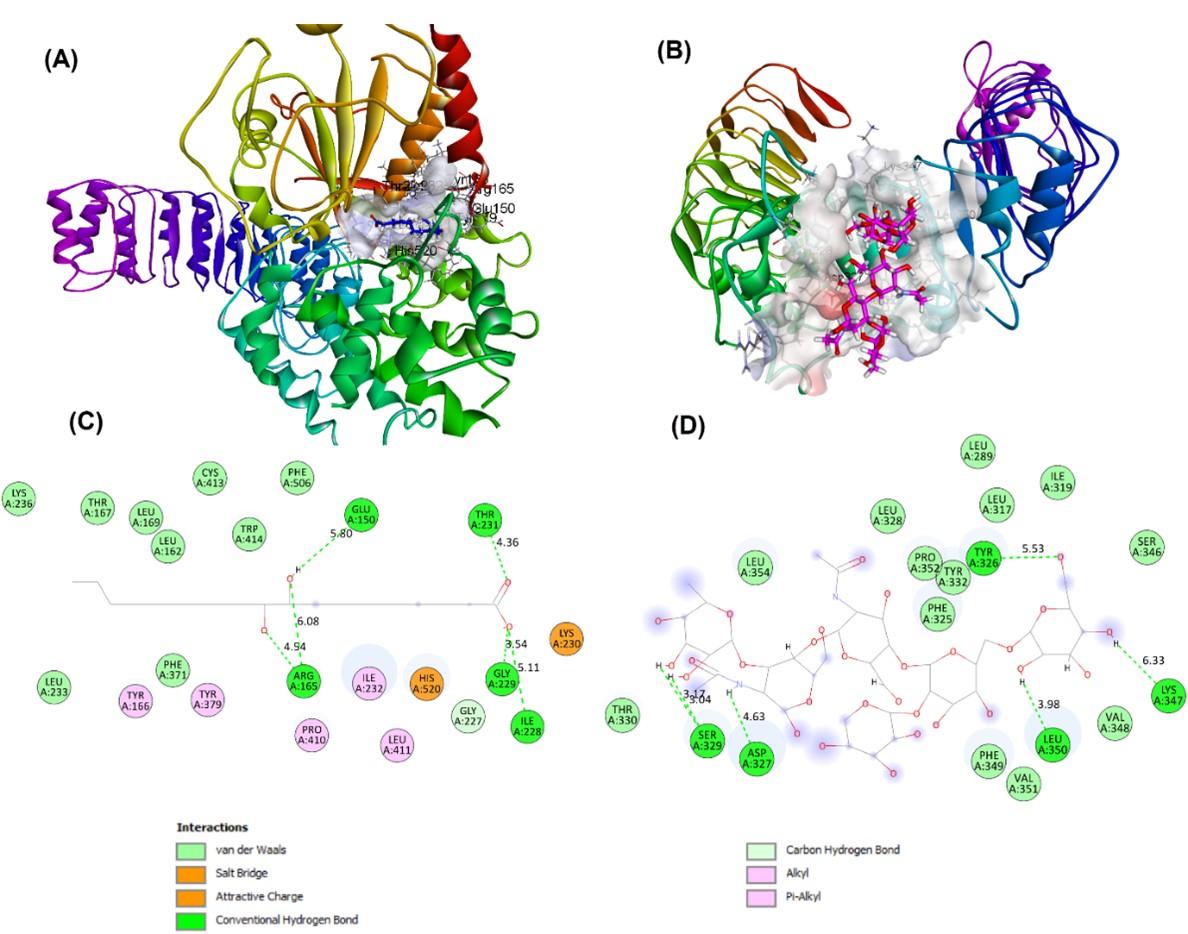
Molecular dynamic simulations
In the current study, MD was used to validate the stability and dynamic behavior of the 89377-NLRP3 and 44263865-TLR2 complexes over a 50 ns period. Figure 7A displays the RMSD values of the apoprotein that fluctuated at the simulation's inception, with the TLR2-44263865 complex showing less variation and fluctuation from the start. However, the TLR2-44263865 complex had a lower RMSD than the apoprotein, indicating its stable nature. Additionally, the root-mean-square fluctuations (RMSF) of both complexes were evaluated to identify the flexibility among amino acid residues. Figure 7B indicates that most residues had lower RMSF, indicating the stable nature of the complex. The radius of gyration of the complexes describes the mobile nature of the complex, with higher Rg correlating with the complex's flexible nature and lower Rg defining the complex's rigid nature. Figure 7C shows that the apoprotein had a higher Rg than the compound complex, indicating the stable nature of the complex. The solvent accessible surface of the complex reveals changes in the protein's surface area. A higher SASA defines the expansion of the surface area, whereas a lower SASA defines the truncated nature of the complex. Figure 7D indicates that the TLR2-44263865 complex had a comparatively lower SASA than the apoprotein, indicating the rigid conformation of the TLR2-44263865 complex.
Furthermore, the dynamic behavior of the NLRP3 and NLRP3-89377 complexes under atomistic simulation conditions was investigated by analyzing the simulation trajectories. Figure 8A indicates that the NLRP3-89377 complex displayed initial expansion at the beginning of the simulation, which may be attributed to its flexible nature. However, both the apoprotein and NLRP3-89377 complex stabilized after 15 ns and maintained stability throughout the simulation, as indicated by the RMSD trend. The RMSF of both complexes were lower than the maximum residues, suggesting their stable nature (Figure 8B). Moreover, the Rg and SASA profiles demonstrated the stable nature of both complexes, with a lower degree of deviation observed (Figures 8 C-D).
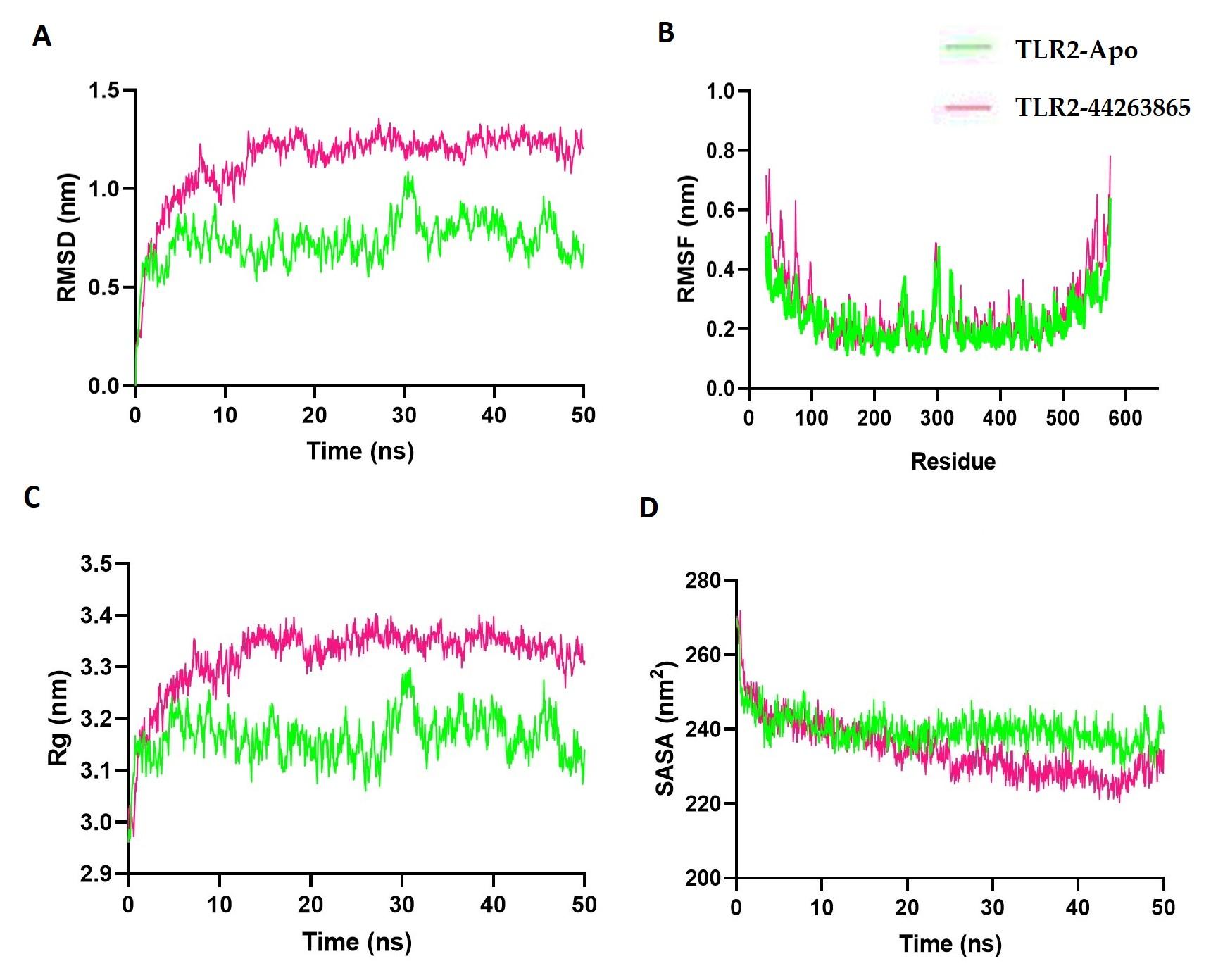
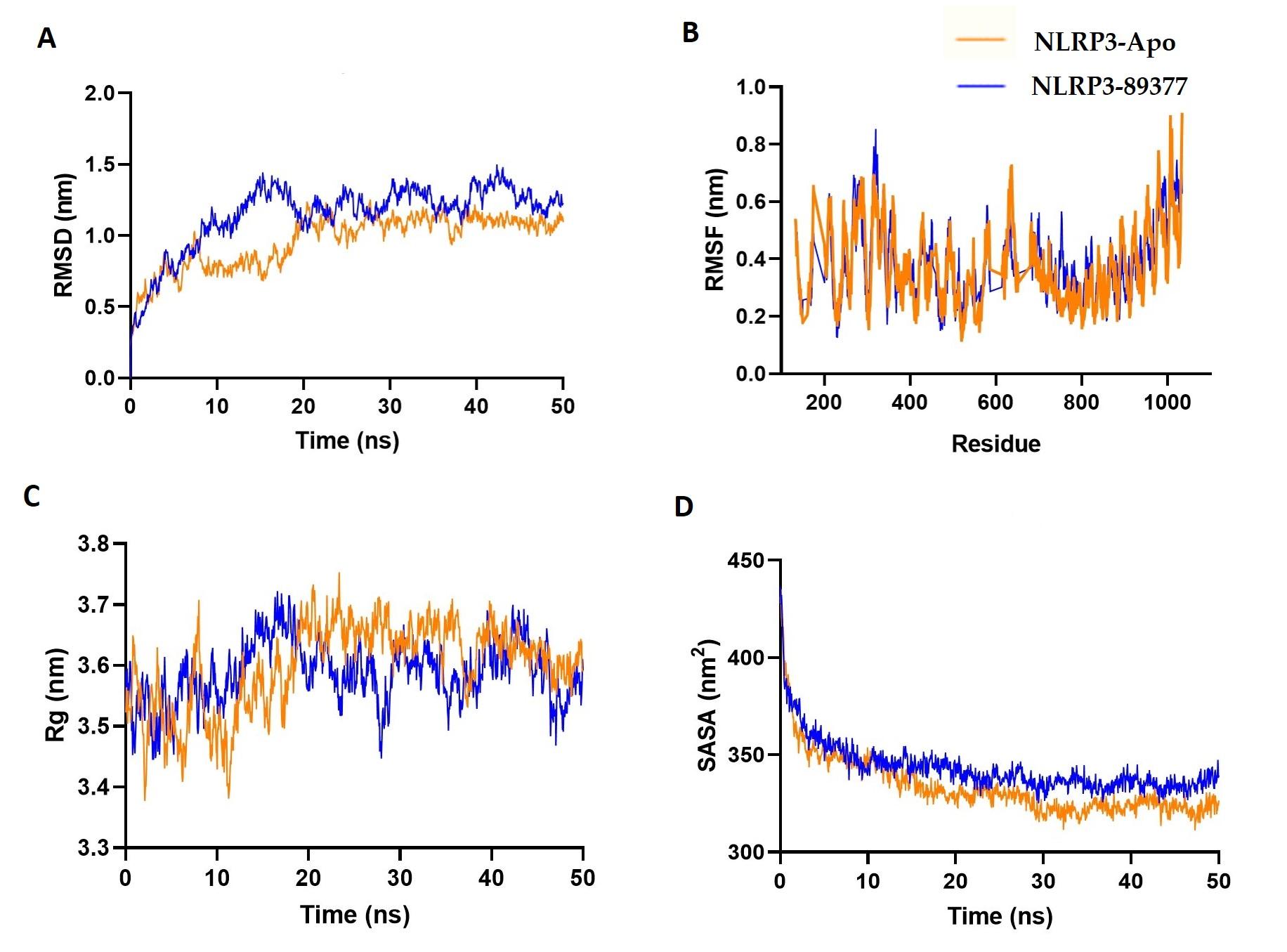
Binding-free energy analysis
Table 3 presents the energies released during the interaction of TLR2-44263865 and NLRP3-89377 complexes between 30 and 50 ns. The BFE of the ligand is proportional to how effectively it binds to the protein, and it is the sum of electrostatic, Van der Waals, polar salvation, and SASA energy. The results indicate that all forms of energy contributed fairly to the interaction of TLR2-44263865 and NLRP3-89377 complexes. The interaction of the TLR2-44263865 complex exhibited a lower BFE (-268.070 ± 19.798 kJ/mol) compared to the NLRP3-89377 complex (-223.975 ± 9.098 kJ/mol).
Table 3. Results of MM-PBSA interaction free binding energies calculation of TLR2-44263865 and NLRP3-89377 complexes between 30 and 50 ns.
DISCUSSION
In the context of a global health crisis, it is of the utmost significance to provide robust health care. Maintaining a well-functioning immune system is an effective strategy for mitigating the risk of exposure to infectious agents [22]. Adequate nutritional intake, encompassing a nutrient-dense diet replete with healthful components sourced from fruits such as pineapple, fulfills the prerequisites for maintaining immune system health. In this investigation, we delved into the immunomodulatory properties exhibited by the secondary metabolites present in both pineapple juice and its fermented counterpart.
The active constituents retrieved from Kanaya's KNApSAcK database and Dr. Duke's Phytochemical and Ethnobotanical databases were utilized to choose bioactive compounds from liquid chromatography-mass spectrometry (LC-MS) findings. The KNApSAcK Core Database comprises 101,500 associations between species and metabolites, encompassing 20,741 species and 50,048 metabolites Dr. Duke's Phytochemical and Ethnobotanical databases facilitate comprehensive plant, chemical, bioactivity, and ethnobotany searches using scientific or vernacular nomenclature [23]. Moreover, an elimination process was conducted to exclude active compounds classified as prodrugs, specifically those with pro-moieties (carrier-linked prodrugs), as these compounds are incapable of directly exhibiting biological activity in the body due to their potential to hinder metabolism and drug absorption via cellular membranes. Pro-moiety consists of carbonate, carbamate, phosphate, amine, ester, amide, and ether groups.
Following the selection phase, 12 bioactive compounds demonstrating high promise were identified as pineapple metabolites with potential immunomodulatory properties. These compounds were found both in the list of compounds generated through data mining and in the LC-MS analysis. The compounds include 1-O-caffeoyl-3-O-feruloylglycerol, ascorbic acid, caffeic acid, citric acid, ferulic acid, linoleic acid, oleic acid, palmitic acid, palmitoleic acid, pantothenic acid, serotonin, and stearic acid. These substances are present in varying concentrations in both pineapple juice (JN) and fermented pineapple juice (F-JN). However, studies have also observed volatile metabolites in freeze-dried pineapple fruit, encompassing γ-lactones, ethyl esters, acetates, acetoxy esters, 2,5-dimethyl-4-hydroxy-3(2H)-furanone, methyl hexanoate, and various alcohols [24]. This can be explained by the variability in metabolite composition, which is influenced by factors such as the type of fruit and the environmental conditions in which it is grown.
Limited data exists concerning the compound 1-O-caffeoyl-3-O-feruloylglycerol, leaving its bioactivity unexplored. Extensive studies have indicated that ascorbic acid possesses antimicrobial attributes, thereby lowering infection risks, along with immunomodulatory effects, especially in higher concentrations [25]. Serotonin (5-hydroxytryptamine; SER) is a physiologically active amine that serves as a well-established neurotransmitter in mammals, modulating mood, sleep, and anxiety. Initially discovered in the legume Mucuna pruriens, serotonin has since been identified in approximately 42 plant species across 20 families [26]. Furthermore, serotonin can affect various immunological processes, such as chemotaxis, leukocyte activation, proliferation, cytokine secretion, anergy, and apoptosis [27].
Apart from the most significant 12 compounds, around 21 derivative compounds emerged in the heatmap. Notably, compounds like caffeic acid, citric acid, ferulic acid, and pantothenic acid appeared in both forms during LC-MS analysis. Comparative abundance data from LC-MS revealed the presence of nearly all compounds in both samples. However, 1-O-caffeoyl-3-O-feruloylglycerol and ethyl vanillin isobutyrate were notably more abundant in the F-JN sample.
The evaluation of a compound's biological or pharmacological activity is imperative during the drug discovery process, as it elucidates the effect of the compound on the target host, which could be either beneficial or detrimental. The probability of a compound being active (Pa) represents the potential of a test compound. The analysis of the potential bioactivity of the metabolite compounds was conducted utilizing the Way2Drug Pass Online webserver. This metric was determined by comparing the structure of the given compounds from pineapple to those with proven efficacy in certain treatments. A compound surpassing a Pa value of 0.7 is anticipated to possess strong immunomodulatory potential due to its close resemblance to a database compound that's been effective in treatment. Conversely, if the Pa value lies between 0.3 and 0.7, the compound has computational activity, albeit with limited similarity to proven effective compounds.
Apart from immunomodulation, pineapple secondary metabolites were investigated for anti-inflammatory and free radical scavenger properties, among others. The study highlights significant secondary metabolites in pineapple with notable prostaglandin-reductase and COX inhibitor activities. COX inhibitors are commonly used for pain, fever, and inflammation management [28]. Utilizing COX-2 inhibitors in the treatment of COVID-19 was safe, as it attenuated the hyper-inflammatory immune response correlated with severe illness [29]. This is attributed to the fact that the coronavirus responsible for severe acute respiratory syndrome (SARS) has been demonstrated to induce COX2 in mammalian cells through both its S and N proteins present in its nucleocapsid [30]. Due to the limited availability of definitive treatments for COVID-19, alternative COX2 inhibition—which is a crucial enzyme in the synthesis of inflammatory cytokines—warrants additional focus and investigation as a potential treatment strategy [31].
The carbohydrate moiety of bromelain (CMB), 1-O-Caffeoyl-3-O-feruloylglycerol, and ferulic acid exhibited remarkable capability in scavenging free radicals. Ascorbic acid, linoleic acid, alpha-linolenic acid, alpha-methylene-gamma-butyrolactone, and geranylacetone all display substantial anti-inflammatory effects. Numerous studies concur that pineapple is an outstanding antioxidant source [32]. One recognized mechanism through which antioxidants impede lipid oxidation is free radical scavenging. This assay is a standard in antioxidant activity research and offers a rapid technique for determining the radical-scavenging capacity of specific compounds or extracts [33].
Pineapple vinegar has the potential to diminish the levels of pro-inflammatory cytokines IL-1β and IL-10. Furthermore, pineapple vinegar exhibits immunostimulatory activity, elevating the levels of CD4+/CD3+, CD8+/CD3+, and both natural killer cell populations (NK1.1+/CD3+ and NK1.1+/CD3), while substantially decreasing the level of macrophages in mouse splenocytes [34]. Pineapple vinegar has also demonstrated the ability to mitigate inflammation in tumors by reducing nitric oxide (NO) and lipid peroxidation levels in tumor homogenate samples. Regular pineapple consumption leads to a decrease in pro-inflammatory cytokines IL-6 and IL- 1β levels in the heart [35].
Drug discovery and development constitute a protracted and costly process, encompassing disease selection, target identification, and validation, lead discovery and optimization, as well as preclinical and clinical trials. Moreover, it requires multidisciplinary, cross-sector collaboration and adherence to regulations [36]. Evaluating a lead compound's chemical absorption, distribution, metabolism, excretion, and toxicity (ADMET) properties is critical in this field [37]. AdmetSAR offers a user-friendly interface for exploring and predicting chemical ADMET profiles. Utilizing the AdmetSAR v.2.0 database, Lipinski's rule and ADMET forecasts were obtained using the CAS registry number (CASRN) and similarity search. Compounds with potential toxicity and/or Lipinski's rule violations are emphasized in bold. Lipinski's rule of 5 (Ro5) distinguishes between drug-like and non-drug-like molecules. It predicts a compound's metabolism outcome based on its resemblance to a drug molecule, with three or more of the following criteria: molecular mass under 500 Daltons, logP below 5, fewer than five hydrogen bond donors, fewer than ten hydrogen bond acceptors, and molar refractivity between 40 and 130 [38].
Identifying compound-target protein interactions is crucial for understanding a compound's mechanism of action. The potential targets of the compounds can be discerned through network analysis. The BINGO analysis conducted using GOlorize provided insights into the role of each compound within biological processes and the associations among target proteins. Furthermore, target proteins linked to reactive oxygen species, immune and inflammatory responses, and oxidative stress were explored, incorporating variables such as stress, degree, betweenness centrality, and closeness centrality. Reactive oxygen species are oxygen-containing molecules that exhibit high reactivity. They can emerge as byproducts during cellular processes and potentially harm cellular components, contributing to disease development. Consequently, target proteins implicated in ROS response are pivotal for upholding cellular balance and averting disease initiation [39]. The immune response safeguards the body from foreign entities like viruses and bacteria, with essential proteins overseeing its balance. Inflammation, a natural reaction to harm or infection, engages immune cells and signaling molecules. Proteins managing this response are pivotal in moderating its impact and averting long-term inflammation. Previous studies reinforce pineapple's potential as an anti-inflammatory, immunomodulatory agent, and infection reducer [14], which aligns with the identified promising proteins in this context. Additionally, pineapple demonstrates antioxidant activity [40,41]
During current public health crises like the COVID-19 pandemic, it has been observed that affected patients may benefit from agents or medications designed to modulate their immune system. These interventions can either enhance innate immune responses or mitigate excessive inflammatory reactions [42]. However, the deployment of targeted immunomodulatory treatments should be approached cautiously, as their effectiveness is dependent on various factors, such as disease progression and the immune status of patients. This is exemplified in cases of severe COVID-19, among other infectious diseases [43].
Moreover, to gain insights into target proteins that have been evaluated for their immunomodulatory roles in the pathological condition such as COVID-19, an analysis was conducted on 25 of the most promising proteins. Based on a literature search, seven of these proteins have demonstrated potential for infectious disease therapy. These include histone acetyltransferase p300 (EP300) [44], prostaglandin G/H synthase 2 (PTGS2) [45], epidermal growth factor receptor (EGFR) [46], nuclear factor erythroid 2-related factor 2 (NFE2L2) [47], Tumor necrosis factor (TNF-α) [48], prostaglandin E synthase (PTGES) [49], and transcription factor p65 (RELA) [44]. Moreover, activation of the inflammasome and pyroptosis may be crucial events that are often overlooked in COVID-19 pathogenesis [50]. By inhibiting inflammasome-mediated pyroptosis in macrophages, abnormal blood clotting could be reduced by preventing the release of tissue factor, a key initiator of blood coagulation cascades [51]. Consequently, although the inflammasome was not detected in any of the 25 target proteins studied in this research, it was taken into account in subsequent analyses.
Several proteins/receptors were chosen for molecular docking analysis, guided by analysis of protein targets from pineapple juice/fermented metabolites. Among them is the nuclear factor kappa B (NF-kB) transcription factor, essential for inflammation mediation and the regulation of innate and adaptive immune functions [52]. Also, toll-like receptor 2 (TLR2) was selected. It is a cell membrane protein identifying foreign agents like viruses, bacteria, and fungi, triggering immune system signals. TLR2 forms partnerships with TLR1 and TLR6, initiating a cascade leading to vital innate immune responses, adaptive immunity development, and protection against pathogen-related immune complications [53]. Tumor necrosis factor alpha (TNF-α), a proinflammatory cytokine, also plays a role in the innate immune response [54]. Additionally, the NLRP3 inflammasome detects a range of pathogen-associated molecular patterns (PAMPs) and danger-associated molecular patterns (DAMPs) produced during viral replication, activating antiviral immune responses and facilitating viral eradication [55]. However, in certain circumstances, overexpression of these proteins can be unfavorable to the body, leading to an attack on the body, as seen during the cytokine storm in COVID-19 patients [56].
The most favorable associations were noted between CMB and TLR2, and between 9,10-dihydroxystearic acid (9,10-DHSA) and NLRP3, highlighted in bold. TLR2 has been linked to COVID-19 severity due to its role in β-coronavirus-triggered inflammation. It identifies the SARS-CoV-2 envelope protein, prompting the release of inflammatory cytokines [57]. TLR2 activation can trigger helpful pro-inflammatory responses that are important in managing infections and eliminating bacteria. Nevertheless, too much TLR2 signalling can result in tissue harm and the advancement of diseases due to an imbalanced production of inflammation [58]. Additionally, it was reported that inflammasomes are activated in response to SARS-CoV-2 infection and are linked to the severity of COVID-19 in patients [59]. Therefore, in severe pathogenic infections, it is suggested that the NLRP3 inflammasome can be targeted [60].
9,10-DHSA, also known as 9,10-dihydroxyoctadecanoic acid, is a hydroxylated fatty acid originating from stearic acid (octadecanoic acid) through the addition of hydroxyl groups at the 9th and 10th carbon positions. This compound belongs to the dihydroxy monocarboxylic acid class, specifically the hydroxyoctadecanoic acid group, and can be referred to as the conjugate acid of 9,10-dihydroxystearate. Bacteria can produce hydroxy fatty acids like 9,10-DHSA through enzymatic reactions, using stearic acid as a substrate for specialized bacterial enzymes that introduce hydroxyl groups at specific locations on the fatty acid chain, such as the 9th and 10th carbon atoms [61]. 9,10-DHSA has been found to exhibit interesting biological properties that could potentially benefit human health. Studies have suggested that this compound may possess anti-inflammatory and antioxidant properties, which could contribute to reducing the risk of chronic diseases such as cancer and cardiovascular disease. Additionally, 9,10-DHSA has shown potential as a treatment for diabetes. In animal studies, DHSA demonstrated an ability to enhance insulin sensitivity and decrease blood glucose levels, suggesting its potential usefulness in managing diabetes [62].
9,10-DHSA has recently gained significant attention due to its potential applications across a range of industries, including cosmetics and pharmaceuticals [63]. As a multifaceted and propitious compound, 9,10-DHSA harbors the potential to revolutionize diverse sectors by proffering innovative and efficacious solutions. Within the realm of cosmetics, this compound could be integrated into an array of formulations, such as emollient creams, lotions, and serums, to augment their efficacy and confer supplementary advantages to end-users. Furthermore, its potent antioxidant properties render it an attractive component for anti-aging and cutaneous protection applications, as it possesses the capacity to mitigate the deleterious impact of reactive oxygen species and environmental stressors on the integumentary system. Pertaining to the pharmaceutical domain, 9,10-DHSA demonstrates promise as an essential constituent in the synthesis of novel drugs and therapeutic agents. Its distinctive physicochemical properties and biological activities may facilitate its pivotal role in addressing an assortment of health-related issues. Potential applications encompass therapies for inflammatory conditions, metabolic dysregulation, and neurodegenerative maladies.
The carbohydrate moiety of bromelain consists of mannose, fucose, xylose, and N-acetylglucosamine (GlcNAc) [64]. According to a study, GlcNAc, a type of sugar molecule, may have the potential to hinder the activity of T-helper 1 (Th1) and T-helper 17 (Th17) cells, which are linked to inflammation and autoimmune diseases [65]. This inhibition could prove beneficial in treating central nervous system disorders such as experimental autoimmune encephalomyelitis. Th1 and Th17 cells usually stimulate immune responses against infections, but their overactivity can contribute to the development of autoimmune disorders like multiple sclerosis. By curbing the production of cytokines that trigger inflammation and are generated by Th1 and Th17 cells, GlcNAc can restrict the activity of these cells and alleviate the symptoms of autoimmune diseases.
In modern drug discovery, molecular dynamics (MD) simulations have gained significant importance and have become prevalent computational tools [66]. Overall, the MD simulations substantiated the stability and dynamic characteristics of the studied complexes. the TLR2-44263865 complex demonstrated enhanced stability, indicated by its lower RMSD and RMSF values, as well as reduced SASA. These findings contribute to a comprehensive understanding of their structural and functional attributes.
Furthermore, the investigation delved into the dynamic behavior of NLRP3 and the NLRP3-89377 complex using atomistic simulations. The analysis of simulation trajectories unveiled that while the NLRP3-89377 complex initially exhibited expansion due to its flexibility, both the apoprotein and the complex reached stability after around 15 ns and maintained this equilibrium throughout. The lower RMSF values in comparison to maximum residues emphasized their stable nature. The Rg and SASA profiles reinforced the stable characteristics of both complexes with minimal deviation. The MD simulations presented a consistent picture of stability across both the TLR2-44263865 complex and the NLRP3-89377 complex. These insights provide valuable knowledge of their behaviors at a molecular level, contributing to a better grasp of their functional roles.
The Molecular mechanics Poisson–Boltzmann surface area (MM-PBSA) approach is employed to calculate interaction free energies and has gained prominence in studying biomolecular interactions [67]. The BFE of the ligand reflects its effectiveness in binding to the protein, encompassing electrostatic, Van der Waals, polar solvation, and SASA energy contributions. Results demonstrate the substantial contribution of all energy components to the TLR2-44263865 and NLRP3-89377 complex interactions. Notably, the TLR2-44263865 complex exhibited a lower BFE (-268.070 ± 19.798 kJ/mol) compared to the NLRP3-89377 complex (-223.975 ± 9.098 kJ/mol). This distinction underscores the potentially stronger binding affinity and stability of the TLR2-44263865 complex.
CONCLUSIONS
In this study, we aimed to analyze the bioactivities of metabolites in pineapple juice/fermented juice with the goal of identifying immunomodulatory lead compounds. Network analysis revealed that target proteins are involved in response to reactive oxygen species, immune response, inflammatory response, and response to oxidative stress. Following screening, molecular docking analysis was performed on selected metabolites with immunomodulatory receptors. Based on the Glide emodel and XP score, the carbohydrate moiety of bromelain (CMB) exhibited the best interaction with TLR2, and 9,10-dihydroxystearic acid with NLRP3 (inflammasome). Molecular dynamics simulations were conducted on these two complexes over a 50 ns time period to investigate their binding affinity for the receptors and to examine the trajectories for RMSD, RMSF, Rg, and SASA. The trajectories were found to be both flexible and stable. Additionally, the low binding free energies obtained by MM-PBSA confirmed the strong molecular interactions between the two complexes. This study provides evidence of a strong interaction between CMB and TLR2, as well as 9,10-dihydroxystearic acid and NLRP3, suggesting that these molecules can be further investigated using experimental studies to confirm their therapeutic efficacy as immunomodulatory agents.
ACKNOWLEDGEMENT
The authors would like to extend their heartfelt appreciation to the Directorate of Research, Technology, and Community Service of the Ministry of Education, Culture, Research, and Technology, Republic of Indonesia, for their generous financial assistance that facilitated research endeavors within the National Competitive Basic Research Scheme throughout the fiscal year 2023 (Main contract number 053/E5/PG.02.00.PL/2023 and derivative contract number 189/UN12.13/LT/2023). We also gratefully acknowledge the support and resources provided by TÜBTAK (Turkish Scientific and Technological Research Council), ULAKBIM (Turkish Academic Network and Information Center), and TRUBA (High Performance and Grid Computing Center) for the molecular docking and molecular dynamics simulations presented in this study.
AUTHOR CONTRIBUTIONS
TET, F, and AY designed outlines and drafted the manuscript. NJN, IC, RI, and AY performed the experiments and analyzed the data. TET, F, DK and IC wrote the initial draft of the manuscript. TET, RI, and TBE reviewed the scientific contents described in the manuscript. All authors read and approved the final submitted version of the manuscript.
CONFLICTS OF INTEREST
There is no conflict of interest among the authors.
SUPPLEMENTARY MATERIALS
Supplementary Table 1. Results of pineapple bioactive compounds data mining from the KNApSAcK database, Supplementary Table 2. Results of data mining of pineapple bioactive compounds from the Dr. Duke database, Supplementary Table 3. Results of PASS Online analysis to determine the immunomodulatory potential of pineapple secondary metabolites, Supplementary Table 4. The activities of prostaglandin-reductase, cyclooxygenase inhibitor, and cyclooxygenase inhibitor and substrate in pineapple secondary metabolites, Supplementary Table 5. Anti-inflammatory, non-steroidal antiinflammatory agent, antiinflammatory steroid, free radical scavenger, interleukin agonist, and interleukin antagonist activities of the secondary metabolites of pineapple, Supplementary Table 6. Results of AdmetSAR analysis to determine the metabolism potential and toxicity of bioactive compounds from pineapple, Supplementary Table 7. Results of Protox II analysis to determine the metabolic potential and toxicity of active compounds of secondary metabolites from pineapple, and Supplementary Table 8. The results of the network analysis using Cytoscape (Supplementary Materials).
References
- [1]Marshall JS, Warrington R, et al. An introduction to immunology and immunopathology. Allergy, Asthma Clin Immunol 2018;14:49.
- [2]Warrington R, Watson W, et al. An introduction to immunology and immunopathology. Allergy, Asthma Clin Immunol 2011;7:S1.
- [3]Marques RE, Marques PE, et al. Exploring the Homeostatic and Sensory Roles of the Immune System. Front Immunol 2016;7:125.
- [4]Kayama H, Takeda K. Regulation of intestinal homeostasis by innate and adaptive immunity. Int Immunol 2012;24:673–80.
- [5]He B, Quan L-P, et al. Dysregulation and imbalance of innate and adaptive immunity are involved in the cardiomyopathy progression. Front Cardiovasc Med 2022;9:973279.
- [6]Blach-Olszewska Z, Leszek J. Mechanisms of over-activated innate immune system regulation in autoimmune and neurodegenerative disorders. Neuropsychiatr Dis Treat 2007;3:365–72.
- [7]McCusker C, Upton J, et al. Primary immunodeficiency. Allergy, Asthma, Clin Immunol Off J Can Soc Allergy Clin Immunol 2018;14:61.
- [8]Chen L, Deng H, et al. Inflammatory responses and inflammation-associated diseases in organs. Oncotarget 2018;9:7204–18.
- [9]Liu Y-Z, Wang Y-X, et al. Inflammation: The Common Pathway of Stress-Related Diseases. Front Hum Neurosci 2017;11:316.
- [10]Mustafa MI, Abdelmoneim AH, et al. Cytokine Storm in COVID-19 Patients, Its Impact on Organs and Potential Treatment by QTY Code-Designed Detergent-Free Chemokine Receptors.
- [11]Chen R, Lan Z, et al. Cytokine Storm: The Primary Determinant for the Pathophysiological Evolution of COVID-19 Deterioration. Front Immunol 2021;12.
- [12]Singh N, Baby D, et al. Inflammation and cancer. Ann Afr Med 2019;18:121–6.
- [13]Walker KA, Le Page LM, et al. The role of peripheral inflammatory insults in Alzheimer’s disease: a review and research roadmap. Mol Neurodegener 2023;18:37.
- [14]Chakraborty AJ, Mitra S, et al. Bromelain a potential bioactive compound: A comprehensive overview from a pharmacological perspective. Life (Basel, Switzerland) 2021;11.
- [15]Rathnavelu V, Alitheen NB, et al. Potential role of bromelain in clinical and therapeutic applications. Biomed Reports 2016;5:283–8.
- [16]Brindha P. Role of phytochemicals as immunomodulatory agents: A review. Int J Green Pharm 2016;10.
- [17]Ruiz Rodríguez LG, Zamora Gasga VM, et al. Fruits and fruit by-products as sources of bioactive compounds. Benefits and trends of lactic acid fermentation in the development of novel fruit-based functional beverages. Food Res Int 2021;140:109854.
- [18]Wu H-M, Zhao C-C, et al. TLR2-Melatonin Feedback Loop Regulates the Activation of NLRP3 Inflammasome in Murine Allergic Airway Inflammation. Front Immunol 2020;11:172.
- [19]Wang L, Hauenstein AV. The NLRP3 inflammasome: Mechanism of action, role in disease and therapies. Mol Aspects Med 2020;76:100889.
- [20]Lipinski CA, Lombardo F, et al. Experimental and computational approaches to estimate solubility and permeability in drug discovery and development settings. Adv Drug Deliv Rev 2001;46:3–26.
- [21]Lipinski CA. Lead- and drug-like compounds: the rule-of-five revolution. Drug Discov Today Technol 2004;1:337–41.
- [22]Yousfi N, Bragazzi NL, et al. The COVID-19 pandemic: how to maintain a healthy immune system during the lockdown - a multidisciplinary approach with special focus on athletes. Biol Sport 2020;37:211–6.
- [23]Lans C, Asseldonk T. Dr. Duke’s Phytochemical and Ethnobotanical Databases, a Cornerstone in the Validation of Ethnoveterinary Medicinal Plants, as Demonstrated by Data on Pets in British Columbia. In: Máthé A, editor., Springer New York; 2020.
- [24]Khakimov B, Mongi RJ, et al. A comprehensive and comparative GC-MS metabolomics study of non-volatiles in Tanzanian grown mango, pineapple, jackfruit, baobab and tamarind fruits. Food Chem 2016;213:691–9.
- [25]Hong JM, Kim JH, et al. Vitamin C is taken up by human T cells via sodium-dependent vitamin C transporter 2 (SVCT2) and exerts inhibitory effects on the activation of these cells in vitro. Anat Cell Biol 2016;49:88–98.
- [26]Ramakrishna A, Giridhar P, et al. Phytoserotonin: a review. Plant Signal Behav 2011;6:800–9.
- [27]Arreola R, Becerril-Villanueva E, et al. Immunomodulatory effects mediated by serotonin. J Immunol Res 2015;2015:354957.
- [28]Zarghi A, Arfaei S. Selective COX-2 inhibitors: A review of their structure-activity relationships. Iran J Pharm Res IJPR 2011;10:655–83.
- [29]Ong SWX, Tan WYT, et al. Safety and potential efficacy of cyclooxygenase-2 inhibitors in coronavirus disease 2019. Clin Transl Immunol 2020;9:e1159.
- [30]Yan X, Hao Q, et al. Nucleocapsid protein of SARS-CoV activates the expression of cyclooxygenase-2 by binding directly to regulatory elements for nuclear factor-kappa B and CCAAT/enhancer binding protein. Int J Biochem Cell Biol 2006;38:1417–28.
- [31]Baghaki S, Yalcin CE, et al. COX2 inhibition in the treatment of COVID-19: Review of literature to propose repositioning of celecoxib for randomized controlled studies. Int J Infect Dis 2020;101:29–32.
- [32]Gómez Herrera MD, Alayón Luaces P, et al. Organic compounds determined at different levels of ripening of the pineapple (Ananas comosus L. Merr.) cv Cayenne in two cultivation systems under subtropical conditions. Int J Fruit Sci 2020;20:371–84.
- [33]Amarowicz R, Pegg RB, et al. Free-radical scavenging capacity and antioxidant activity of selected plant species from the Canadian prairies. Food Chem 2004;84:551–62.
- [34]Mohamad NE, Abu N, et al. Apoptosis and metastasis inhibitory potential of pineapple vinegar against mouse mammary gland cells in vitro and in vivo. Nutr Metab (Lond) 2019;16:49.
- [35]Seenak P, Kumphune S, et al. Pineapple consumption reduced cardiac oxidative stress and inflammation in high cholesterol diet-fed rats. Nutr Metab (Lond) 2021;18:36.
- [36]Chen J, Luo X, et al. Drug discovery and drug marketing with the critical roles of modern administration. Am J Transl Res 2018;10:4302–12.
- [37]Guan L, Yang H, et al. ADMET-score - a comprehensive scoring function for evaluation of chemical drug-likeness. Medchemcomm 2019;10:148–57.
- [38]Lipinski CA. Lead-and drug-like compounds: the rule-of-five revolution. Drug Discov Today Technol 2004;1:337–41.
- [39]Yang S, Lian G. ROS and diseases: role in metabolism and energy supply. Mol Cell Biochem 2020;467:1–12.
- [40]Hossain MA, Rahman SMM. Total phenolics, flavonoids and antioxidant activity of tropical fruit pineapple. Food Res Int 2011;44:672–6.
- [41]Lu X-H, Sun D-Q, et al. Physico-chemical properties, antioxidant activity and mineral contents of pineapple genotypes grown in china. Molecules 2014;19:8518–32.
- [42]Razmi M, Hashemi F, et al. Immunomodulatory-based therapy as a potential promising treatment strategy against severe COVID-19 patients: A systematic review. Int Immunopharmacol 2020;88:106942.
- [43]Zhang Y, Chen Y, et al. Immunomodulation for Severe COVID-19 Pneumonia: The State of the Art. Front Immunol 2020;11:2782.
- [44]Salgado-Albarrán M, Navarro-Delgado EI, et al. Comparative transcriptome analysis reveals key epigenetic targets in SARS-CoV-2 infection. Npj Syst Biol Appl 2021;7:21.
- [45]Chen JS, Alfajaro MM, et al. Cyclooxgenase-2 is induced by SARS-CoV-2 infection but does not affect viral entry or replication. BioRxiv Prepr Serv Biol 2020.
- [46]Venkataraman T, Coleman CM, et al. Overactive epidermal growth factor receptor signaling leads to increased fibrosis after severe acute respiratory syndrome coronavirus infection. J Virol 2017;91.
- [47]McCord JM, Hybertson BM, et al. Nrf2 Activator PB125<sup>®</sup> as a Potential Therapeutic Agent Against COVID-19. BioRxiv 2020:2020.05.16.099788.
- [48]Robba C, Battaglini D, et al. Multiple organ dysfunction in SARS-CoV-2: MODS-CoV-2. Expert Rev Respir Med 2020;14:865–8.
- [49]Gordon DE, Jang GM, et al. A SARS-CoV-2 protein interaction map reveals targets for drug repurposing. Nature 2020;583:459–68.
- [50]Yap JKY, Moriyama M, et al. Inflammasomes and pyroptosis as therapeutic targets for COVID-19. J Immunol 2020;205:307–12.
- [51]Wu C, Lu W, et al. Inflammasome activation triggers blood clotting and host death through pyroptosis. Immunity 2019;50:1401-1411.e4.
- [52]Liu T, Zhang L, et al. NF-κB signaling in inflammation. Signal Transduct Target Ther 2017;2:17023.
- [53]de Oliviera Nascimento L, Massari P, et al. The role of TLR2 in infection and immunity. Front Immunol 2012;3:79.
- [54]Olmos G, Lladó J. Tumor necrosis factor alpha: A link between Nneuroinflammation and excitotoxicity. Mediators Inflamm 2014;2014:861231.
- [55]Zhao C, Zhao W. NLRP3 inflammasome—A key player in antiviral responses. Front Immunol 2020;11:211.
- [56]Melo AKG, Milby KM, et al. Biomarkers of cytokine storm as red flags for severe and fatal COVID-19 cases: A living systematic review and meta-analysis. PLoS One 2021;16:e0253894.
- [57]Zheng M, Karki R, et al. TLR2 senses the SARS-CoV-2 envelope protein to produce inflammatory cytokines. Nat Immunol 2021;22:829–38.
- [58]Simpson ME, Petri WAJ. TLR2 as a Therapeutic Target in Bacterial Infection. Trends Mol Med 2020;26:715–7.
- [59]Rodrigues TS, de Sá KSGG, et al. Inflammasomes are activated in response to SARS-cov-2 infection and are associated with COVID-19 severity in patients. J Exp Med 2020;218.
- [60]Freeman TL, Swartz TH. Targeting the NLRP3 inflammasome in severe COVID-19. Front Immunol 2020;11:1518.
- [61]Kaprakkaden A, Srivastava P, et al. In vitro synthesis of 9,10-dihydroxyhexadecanoic acid using recombinant Escherichia coli. Microb Cell Fact 2017;16:85.
- [62]Yu X, Zhang Y, Liu et al. Effects of 9,10-dihydroxysteatic acid on glucose metabolism in KKAy mice. Wei Sheng Yan Jiu 2010;39:423–5.
- [63]Razmah G, Afida I, et al. Biodegradation of Palm-based 9,10-Dihydroxystearic Acid (DHSA)in aquatic environments. Sains Malaysiana 2015;44:1263–1268.
- [64]Ishihara H, Takahashi N, et al. Complete structure of the carbohydrate moiety of stem bromelain. An application of the almond glycopeptidase for structural studies of glycopeptides. J Biol Chem 1979;254 21:10715–9.
- [65]Grigorian A, Araujo L, et al. N-acetylglucosamine inhibits T-helper 1 (Th1)/T-helper 17 (Th17) cell responses and treats experimental autoimmune encephalomyelitis. J Biol Chem 2011;286:40133–41.
- [66]Salo-Ahen OMH, Alanko I, et al. Molecular dynamics simulations in drug discovery and pharmaceutical development. Processes 2021;9.
- [67]Kumari R, Kumar R, et al. G-mmpbsa -A GROMACS tool for high-throughput MM-PBSA calculations. J Chem Inf Model 2014;54:1951–62.