Interaction of epitopic missense variants of VEGFA with therapeutic monoclonal antibodies
Abstract
Vascular Endothelial Growth Factor A (VEGFA) is a glycoprotein that mediates various biological processes, including angiogenesis, vascular permeability, and cellular migration. Aberrant VEGFA signaling is also one of the hallmarks of many types of cancer and has been implicated in various ophthalmological conditions such as diabetic macular edema and age-related macular degeneration. Consequently, a number of therapeutic monoclonal antibodies (mAb) targeting VEGFA have been developed and are widely used to treat these conditions. Bevacizumab (BVZ) and Ranibizumab (RBZ) are two such antibodies that are commercially available and used to treat various cancers and ophthalmological conditions. Nevertheless, a very high rate of non-responsiveness to these mAb treatments has been reported. Therefore, it is important to predict the response to these therapeutic mAb treatments in patients in a personalized approach. This study was aimed at analyzing the impacts of missense variants in the respective VEGFA epitopes for these two therapeutic anti-VEGFA mAbs (BVZ and RBZ) on their interaction with VEGFA through the use of multiple in silico tools. Three missense variants (VEGFAR82W, VEGFAR82Q, and VEGFAG92R) in VEGFA epitopes appear to significantly destabilize VEGFA-BVZ interaction, while only two variants (VEGFAR82W and VEGFAR82Q) affect the interaction of VEGFA with RBZ. The VEGFAR82W variant may be pathogenic as well. These missense variants may play roles in the observed heterogeneous response to anti-VEGFA mAb treatments in patients and, therefore, may be used as pharmacogenetic markers for the prediction of responses before administration, and thus for the improvement of therapeutic outcomes.
INTRODUCTION
Vascular Endothelial Growth Factor A (VEGFA) is a glycoprotein that belongs to the VEGF gene family and is involved in many cellular and physiological processes such as vasculogenesis, angiogenesis, migration, and mitogenesis of endothelial cells (EC) [1-3]. VEGFA is also known as vascular permeability factor (VPF) due to its role in increasing vascular permeability and eliciting inflammation [4]. Expression of VEGFA occurs in most human cell types such as endothelial cells, blood cells, cardiomyocytes, etc. [4, 5]. VEGFA gene consists of eight exons, and multiple isoforms of the glycoprotein are produced through alternative splicing [5]. VEGFA exerts its pleiotropic effect on cellular processes by binding to two homologous cell surface receptors: Vascular Endothelial Growth Factor Receptor 1 (VEGFR1, also known as Flt-1) and Vascular Endothelial Growth Factor Receptor 2 (VEGFR2, also known as Flk-1) [4, 6]. The affinity of VEGFR1 for VEGFA is one order higher than the affinity of VEGFR2 for VEGFA. However, the kinase activity is 10-fold greater in VEGFR2 compared to VEGFR1 [3]. Therefore, VEGFR2 is considered the more prominent of the two receptors in transducing VEGFA signal [7].
VEGFA is overexpressed in many types of malignant tumors, and its overexpression, which stimulates angiogenesis, is considered one of the hallmarks of various cancers [8, 9]. Overexpression and subsequent secretion of VEGFA by hypoxic tumor cells and stroma in the tumor microenvironment promotes metastasis and correlates with poor prognosis [10-12]. VEGFA signaling has also been implicated in function and maintenance of cancer stem cells [7]. Aberrant VEGFA signaling has also been implicated in a wide array of pathological conditions such as corneal inflammation and other inflammatory disorders, intraocular neovascular syndrome, and brain edema [12, 13]. In various studies, it has been shown that treatment with VEGFA blocking monoclonal antibodies (mAbs) that disrupt the receptor-VEGFA interaction, remarkably suppresses tumor growth and progression through inhibition of pathological angiogenesis [10, 13]. Two of the currently available FDA approved anti-VEGFA therapeutic mAbs are Bevacizumab (BVZ) and Ranibizumab (RBZ) [14]. BVZ, the first FDA-approved mAb targeting VEGFA, is a humanized mAb that specifically binds to and inhibits the signaling function of circulating, soluble VEGFA isoforms [15]. BVZ is a recombinant therapeutic mAb, whose 93% sequences came from human IgG1 antibody and the remaining 7%, including the complementarity determining regions (CDRs), from murine mAbA. 4.6.1. [16, 17]. Although BVZ was initially used to treat metastatic colorectal cancer (mCRC), it is currently being used in the treatment of non-squamous non-small cell lung cancer (NSCLC), renal cell carcinoma (RCC), Glioblastoma multiforme (GBM), and metastatic cervical cancer (mCC) in combination with other anti-cancer drugs [10]. RBZ, in contrast, is used in the treatment of all forms of diabetic retinopathy including diabetic macular edema (DME), age-related macular degeneration (AMD), and myopic choroidal neovascularization (CNV) [10, 18, 19]. RBZ (~48 kDa) is also based on humanized IgG1 antibody of κ isotype like BVZ (~149 kDa), nevertheless, it is composed of only the antigen binding fragment (Fab)that binds to all biologically active forms of VEGFA [18, 20, 21].
Therapeutic mAbs like BVZ and RBZ are, in general, quite expensive compared to chemotherapeutic drugs [17, 22]. Due to the lower cost of BVZ compared to RBZ, it is more commonly used as off-label therapy in ophthalmological conditions, and it is one of the highest selling mAbs in the world in terms of revenue [22, 23]. In spite of their proven efficacy in clinical trials, their effectiveness is often limited due to a high rate of non-responsiveness [17, 24, 25]. For instance, in case of BVZ, overall response is between 20% and 60% depending on the cancer types resulting in high proportion of non-responsiveness [17, 24]. The rate of non-responsiveness to RBZ is 27% in retinopathy of prematurity (ROP) [25]. Besides, it has been shown that the race of the patient may have a role in responsiveness to RBZ treatment [26]. As a result, determining the underlying causes of non-responsiveness and predicting patient responses to these therapeutic mAbs are critical. Although some studies have reported an association between variants of VEGFA and response to BVZ and RBZ treatment in various cancers and ophthalmological diseases, respectively, much is not known about the impacts of these VEGFA variants on interaction with BVZ and RBZ [27-30]. It was reported that mCRC patients, who are carriers of VEGFA rs699947 and rs1570360 variants, had better clinical outcomes compared to the wildtype variant carriers when treated with bevacizumab, and this difference in prognosis might be attributed to the changes in the affinity of bevacizumab to these VEGFA variants [27, 31]. In addition, a previous computational study of the epitopic missense variants of tissue necrosis factor alpha (TNFA) reported significant destabilization of TNFA-mAb interactions due to some missense variants [32]. In this study, the effects of missense epitopic variants of VEGFA on its interactions with BVZ and RBZ were investigated using in silico tools. The potential pathogenic association of destabilizing epitopic VEGFA variants was also predicted by analyzing their interactions with VEGFR2, the more prominent of the two VEGFA receptors.
MATERIALS AND METHODS
Identification of missense variants in the VEGFA epitopes of the approved anti-VEGFA mAbs
The list of approved anti-VEGFA mAbs (BVZ and RBZ) was retrieved from the Therapeutic Structural Antibody Database (Thera-SabDab) [33]. Using a literature search, epitopic residues critical for VEGFA binding to BVZ and RBZ were identified [31, 34]. The Ensembl Genome Browser was used to collect missense variants at these VEGFA epitopic sites (release 104) [35].
Assessment of the effect of the epitopic missense variants on VEGFA structure and VEGFA-mAb interactions
X-ray crystallographic structures of the VEGFA-BVZ (PDB ID: 1BJ1) and VEGFA-RBZ (PDB ID: 1CZ8) complexes were retrieved from the Protein Data Bank (PDB) [36]. As each of these two structures contained two VEGFA molecules and more than one antibody heavy and light chains, these required tailoring for analyzing interactions between a single epitope and a single Fab fragment. After retaining H (BVZ heavy chain), L (BVZ light chain), and W (VEGFA molecule) from the VEGFA-BVZ complex and H (RBZ heavy chain), L (RBZ light chain), and W (VEGFA molecule) from the VEGFA-RBZ, all the other chains and non-amino acid chemical residues were deleted using UCSF Chimera 1.15rc [37]. The edited VEGFA-BVZ and VEGFA-RBZ complexes were used as input in Missense3D [38] to analyze structural changes resulting from the missense variants as well as in mCSM-PPI2 [39], SAAMBE-3D [40], MutaBind2 [41], and BeAtMuSiC (V1.0) [42] to predict the impacts of the epitopic missense variants on interactions between VEGFA epitope and each of the mAbs.
3D modeling and calculation of stabilizing energy of VEGFA-mAb complexes
The amino acid sequence of human VEGFA (UniProt Accession Number: P15692) was collected from the UniProt knowledgebase (UniProtKB) [43]. The signal peptide comprising the first 26 residues of the retrieved sequence was deleted. In addition, the residues at the variant sites were substituted. Amino acid sequences of the heavy and light chains of BVZ and RBZ were retrieved from Thera-SabDab [33]. 3D models of VEGFA-mAb complexes (both wild type and mutant) were formed through the SWISS-MODEL [44] server using the previously customized structures as templates. UCSF Chimera 1.15rc [37] was used to rename the chains in the server generated models to H (antibody heavy chains), L (antibody light chains), and A (VEGFA molecule). The strength or stabilization energy of the interaction between VEGFA and mAbs was calculated using the PPcheck web server [45]. PyMOL 2.5.4 was used to study the interaction interface of VEGFA-mAb complexes, utilizing 3D models produced by SWISS-MODEL [44] as input.
Exploration of the interface, interactions, and interacting residues of VEGFA and mAbs
Areas of interacting surfaces as well as 2D maps of interactions between VEGFA and each of the H and L chains of mAbs were analyzed with the iCn3D structure viewer [46] with the default parameters. Using Arpeggio Web Server [47], the number of bonds and interactions, including, hydrogen bonds (H-bonds), weak H-bonds, ionic and van der Waals interactions were calculated. SWISS-MODEL generated 3D models of VEGFA-mAb complexes were used as input in both the web servers.
Analysis of pathogenicity of the missense epitopicvariants of VEGFA
Information regarding the deleteriousness of the epitopic missense variants was retrieved from PolyPhen2 and SIFT at the Ensembl Genome Browser (release 104) [35] as well as from Meta-SNP [48] and PredictSNP v1.0 [49] web servers. In addition, the association of selected missense variants with diseases was searched for using PhenoScanner v2 [50] and DisGeNET [51] databases.
Assessment of the influence of the missense variants on interactions between VEGFA and its receptor VEGFR2
In order to analyze the impacts of the missense variants of VEGFA at the epitopic sites for relevant mAbs on interactions between VEGFA and its receptor (VEGFR2), X-ray crystallographic structure of a single VEGFA molecule in complex with domains 2 and 3 of VEGFR2 (PDB ID: 3V2A) was retrieved from the PDB [36]. These two domains mediate the binding of VEGFA to VEGFR-2 [52]. This structure was used as input in mCSM-PPI2 [39], SAAMBE-3D [40], and MutaBind2 [41] for predicting the effect of the missense variants of VEGFA on binding of VEGFA to VEGFR2.
RESULTS
Missense variants in the epitope of VEGFA for anti-VEGFA mAbs
The amino acid residue composition of the VEGFA epitopes for BVZ [34] and RBZ [31] was retrieved through a literature review (Table 1). Five missense variants were found in the epitopes for both BVZ and RBZ according to the data collected through the Ensembl Genome Browser (release 104) [35].
Table 1. Epitopes of VEGFA for Bevacizumab and Ranibizumab.
Impacts of VEGFA epitopic missense variants on binding affinities of anti‑VEGFA therapeutic mAbs for VEGFA
Two distinct approaches were used to predict how epitopic VEGFA missense variants influence interaction between VEGFA and therapeutic mAbs (BVZ and RBZ). In the first approach, the relative changes in binding affinities (ΔΔG = ΔGmutant − ΔGwild-type) caused by the selected variants were estimated using crystallographic structures obtained from the PDB [36] with four different tools: mCSM-PPI2 [39], SAAMBE-3D [40], MutaBind2 [41], and BeAtMuSiC (V1.0) [42]. In the second approach, the SWISS-MODEL [44] server was used to generate 3D models of VEGFA-mAb complexes (both wild type and mutant), and then PPcheck [45] was used to determine the total stabilizing energies of these VEGFA-mAb complexes. Since, substantial alterations in antibody binding affinity in the case of antigen-antibody interactions is frequently considered to have |∆∆G| > 1.0 kcal/mol (4.18 kJ/mol) [53, 54], this value was considered to correlate with substantial decline in binding affinity in all cases. In this study, missense variants were considered to be significantly destabilizing in the context of VEGFA-mAb interaction only when the ∆∆G values were greater than 1.0 at least with three tools.
The variant G92R (rs1456457746) (excluding the signal peptide consisting of 26 amino acid residues) of VEGFA was predicted by all four tools to reduce (∆∆G > 1.0) the binding affinity of BVZ for VEGFA (Table 2). Another variant, R82Q (rs367757959), was also estimated to significantly destabilize the VEGFA-BVZ interaction by three tools (mCSM-PPI2, SAAMBE-3D, and MutaBind2). The total binding energies (predicted by PPcheck) for the VEGFAR82Q-BVZ heavy chain (H chain) complex were also significantly lower (15.17 kJ/mol) compared to wild-type VEGFA, implying that the R82Q variant severely weakens the interaction between VEGFA and BVZ. All four tools, which were used for estimating the differences in binding affinities caused by the variants, predicted that the G92R variant significantly impaired (∆∆G >1.0 kcal/mol) the interaction between BVZ and VEGFA; however, this result was not corroborated with the predictions of PPcheck (Table 2). Although only two tools (SAAMBE-3D and MutaBind2) predicted lower binding affinities due to the R82W variant, this variant might have some destabilizing effects since PPcheck estimated a notable decrease (9.31 kJ/mol compared to wildtype) in the total binding energies of the VEGFAR82W and BVZ heavy chain complexes (Table 2).
In case of VEGFA-RBZ interactions, the R82W and R82Q variants were predicted by three (mCSM-PPI2, SAAMBE-3D, and MutaBind2) out of the four tools used to significantly alter binding affinities (∆∆G >1.0 kcal/mol) and, therefore, weaken VEGFA-RBZ interactions (Table 3). However, these findings for both the variants were not substantiated by the predictions of PPcheck since PPcheck estimated an increase in total binding energies (9.08 kJ/mol for the R82W variant and 7.70 kJ/mol for the R82Q variant compared to wildtype) for both the VEGFAR82W-RBZ H chain complex and the VEGFAR82Q-RBZ H chain complex (Table 3). The other variants were predicted not to have significant destabilizing effects (with three or more tools) on the interaction between VEGFA and RBZ (Table 3).
Table 2. Effects of epitopic missense variants on VEGFA-Bevacizumab interaction.
Table 3. Effects of epitopic missense variants on VEGFA-Ranibizumab interaction.
Interaction of epitopic missense variants of VEGFA with anti-VEGFA mAbs and VEGFR2
The Arg82 residue of wild-type VEGFA contacted with (cut off value 4Å) the Gly104 residue of the H chain of BVZ. However, in VEGFAR82Q variant the Gln82 residue was no longer in contact with Gly104 residue of BVZ H chain (Figures 1A and 1C). The π-cation interaction with Tyr102 of the BVZ H chain caused no structural change in VEGFA (Figure 3A). In addition, there were H-bonds between Tyr45 of VEGFAWT and Ser106 of BVZ H chain (Figure 3A). However, due to structural change in VEGFAR82W variant, H bonds between Trp82 (VEGFA) and Ser106 (BVZ H chain) were lost (Figure 3B). Furthermore, the π-cation interaction between Trp82 (VEGFA) and Tyr102 (BVZ H chain) was converted into an H-bond, which was 2.5 to 5 times weaker than the cation-pi interaction (Figure 3B) [55]. Moreover, the conformational change due to VEGFAR82W variant was predicted to trigger a clash between VEGFA and BVZ, further affecting the stability of the VEGFA-BVZ H chain complex (Table 4). With both VEGFAR82W and VEGFAR82Q variants, all the ionic interactions between VEGFA and BVZH and L chains were lost, which were present in the VEGFAWT and BVZ complex, which further weakened the interaction between VEGFA and the BVZ H chain (Table 4). The Arg92 residue of the VEGFAG92R variant were in close contact with the Pro100, Ser105, Ser106, and His107 residues of the BVZ H chain, which were more than 4Å residues away from the Gly92 residue of the VEGFAWT (Figure 1A and 1F). Additionally, the H-bonds between Arg82 of VEGFA and Ser106 of the BVZ H chain, as well as between Ile91 of VEGFA and Tyr102 of the BVZ H chain, were lost due to the VEGFAG92R variant (Figures 3A and 3F). These changes in proximity and interactions have a significant impact on the stability of the VEGFA-BVZ complex as predicted with mCSM-PPI2, SAAMBE-3D, MutaBind2, and BeAtMuSiC V1.0 tools. A significant increase (98.57 Å2 compared to wild-type) in buried surface area in VEGFA-BVZ H chain interface was predicted to be caused by this variant. The other variants caused no structural changes and a significant change in the intermolecular bonding pattern (Figure 2) (Table 4).
In the VEGFAWT-RBZ H chain complex, the distance between Arg82 of VEGFA and Thr105 of the RBZ H chain was greater than 4 Å. However, in the VEGFAR82W variant, the two residues, Trp82 of VEGFA and Thr105 of RBZ H chain, came in close contact due to a structural change, which was also predicted to cause a clash between VEGFA and the RBZ H chain, destabilizing the VEGFA-RBZ complex (Figures 2A and 2B). The structural change was also accounted for by the conversion of a cation-pi interaction into a much weaker (2.5 to 5 times weaker) H bond between Trp82 of VEGFA and Tyr102 of the RBZ H chain (Figures 4A and 4B) [55]. Arg82 of VEGFAWT was in close proximity of Gly104 of RBZ H chain; nevertheless, in the VEGFAR82Q variant Gln82 lost contact with Gly104 of RBZ H chain. Although no structural change was predicted for the variant, a new H bond formed between Gln82 of VEGFA and Ser106 of the RBZ H chain which was not present in the VEGFAWT-RBZ H chain complex (Figures 4A and 4C). Additionally, the cation-pi interaction between Arg82 of VEGFA and Tyr102 of the RBZ H chain was lost due to VEGFAR82Q variant (Figure 4A and 4C). All of the salt bridges present in the VEGFAWT-RBZ L chain complex were lost due to both VEGFAR82W and VEGFAR82Q variants (Table 4). No conformational change or alteration in interactions was predicted for the other variants.
None of the six variants of VEGFA (VEGFAR82W, VEGFAR82Q, VEGFAI83L, VEGFAQ87R, VEGFAG88S, and VEGFAG92R), three of which significantly destabilized VEGFA-mAb interaction, were predicted to cause significant destabilization (∆∆G >1.0 kcal/mol) of VEGFA-VEGFR2 interactions.
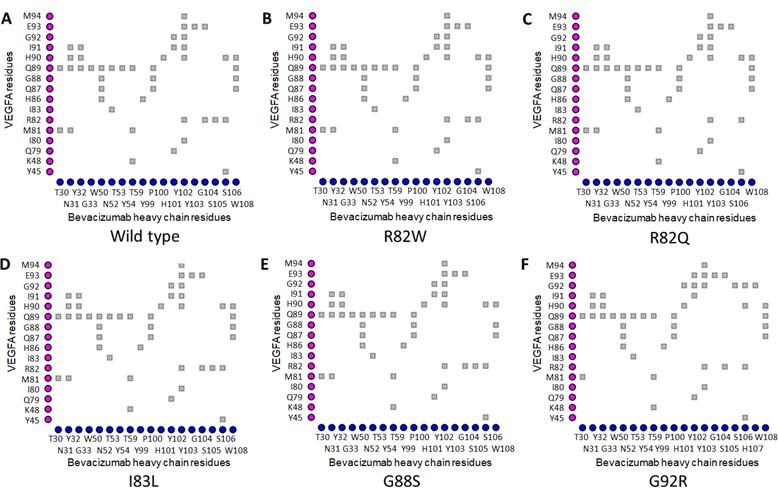
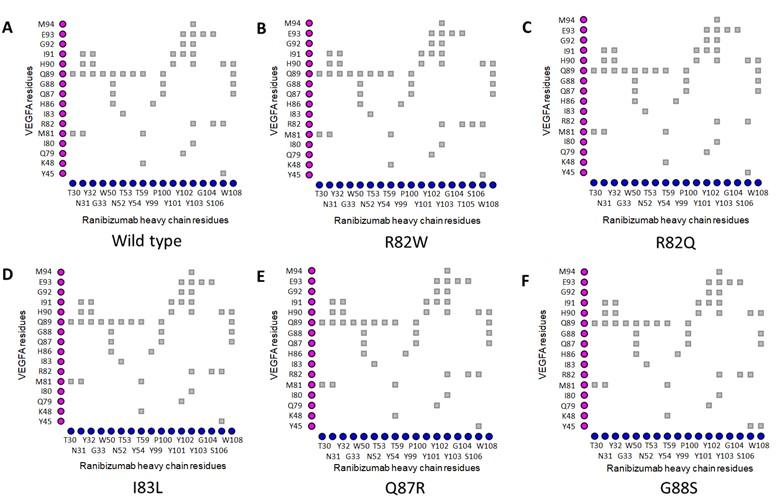
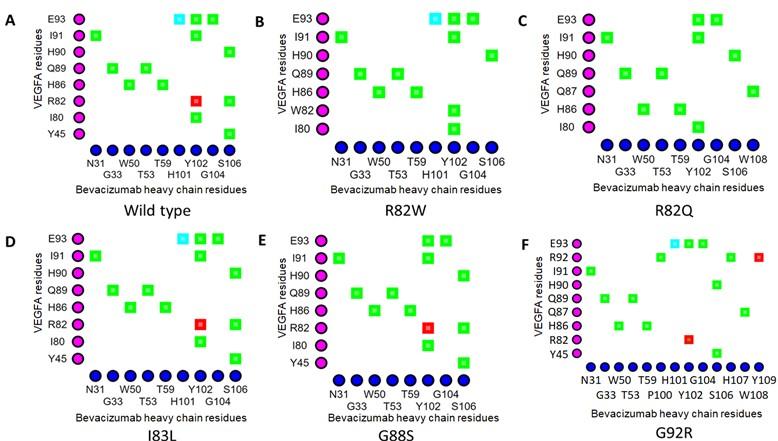
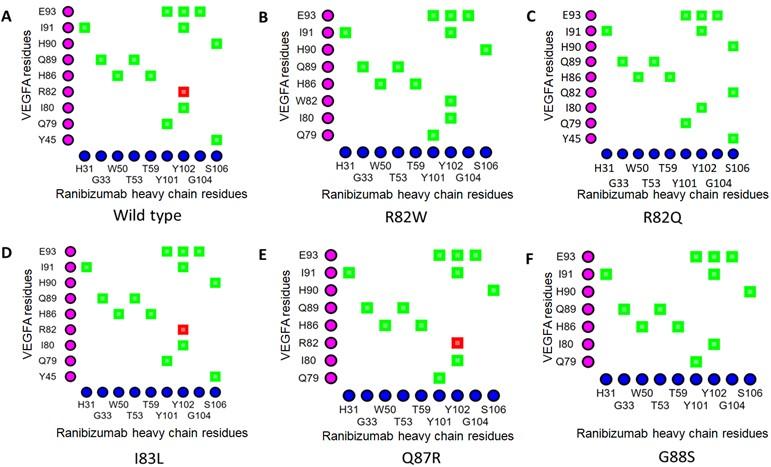
Table 4. Impacts of selected epitopic missense variants on VEGFA structure and VEGFA-mAb interactions.
Pathogenicity of epitopic missense variants of VEGFA
PolyPhen2 and SIFT at Ensembl Genome Browser (release 104) [35], Meta-SNP [48] and PredictSNP v1.0 [49] web servers were used to make predictions about the deleteriousness of the epitopic missense variants of VEGFA that were predicted to have destabilizing effects on VEGFA-BVZ or VEGFA-RBZ interactions. Only rs114262569 (VEGFAR82W) was predicted to have detrimental effects by all four tools (Table 5). The missense variant rs367757959 (VEGFAR82Q) was predicted to have a deleterious impact by only PredictSNP v1.0 [49] while the remaining three tools showed no harmful effect for this variant (Table 6). Besides, when the variants were searched for their association with disease using the PhenoScanner v2 [50] and DisGeNET [51] databases, only the variant rs1284410244 (VEGFAQ87R) was found to be associated with disease, namely fibrosarcoma (according to the DisGeNET database only), while for all other missense variants, no association with disease was found in either of the databases.
Table 5. Predicted pathogenicity of the selected missense variants of VEGFA epitope.
Table 6. Effects of epitopic missense variants of VEGFA on VEGFA-VEGFR2 interaction.
DISCUSSION
The primary goal of this study was to analyze the impacts of missense variants of VEGFA epitopes on their interaction with therapeutic anti-VEGFA mAbs for the purpose of identifying any missense variant that can be a good predictor of responsiveness to these therapeutic mAbs. The four tools (mCSM-PPI2, SAAMBE-3D, MutaBind2, and BeAtMuSiC V1.0) that were used for assessing the impacts of epitopic missense variants on the binding affinities of therapeutic mAbs (BVZ and RBZ) to VEGFA work on different algorithms to predict the change in binding affinities (∆∆G). mCSM-PPI2 is a web server based on novel machine learning (ML) technique that uses graph-based structural signatures, known as mCSM (mutation Cutoff Scanning Matrix), to accurately predict the alterations in binding affinity of protein-protein interactions resulting from missense variants [39]. Pre-existing tools were shown to be outperformed by mCSM-PPI2 [39]. MutaBind2 employs a minimization protocol and a scoring function based on seven features including the term describing the thermodynamic stability of the protein complex and each monomer as well as the interactions of proteins with the solvent [41]. It can predict the effects of both single and multiple mutational events on the binding affinity of proteins [41]. SAAMBE-3D, a relatively new development of the SAAMBE (Single Amino Acid Mutation change of Binding Energy) method, is based on ML and utilizes a combination of molecular mechanics/Poisson Boltzmann surface area (MM/PBSA) and knowledge-based terms to estimate alterations in binding affinities, as well as, dielectric constant of each of the amino acids to simulate the structural flexibility caused by mutation [40]. SAAMBE-3D was shown to be more effective than mCSM-PPI in predicting changes in binding affinities induced by mutation [40]. BeAtMuSiC v1.0 predicts ∆∆G of protein-protein interaction (PPI) based on a set of statistical potentials obtained from well-characterized protein structures, stability of the protein complex, and integrates the impacts of the mutation on the strength of the interactions between proteins at the interface [42]. PPcheck applies docking algorithm and can differentiate native-like and non-native-like docking poses generated by the algorithm [45]. The use of many tools that rely on different working principles may yield conflicting outcomes. But using a number of tools together helps figure out the effects of missense variants more accurately and with more certainty [56]. All of the missense variants were further analyzed to predict their impacts on VEGFA structure and on VEGFA-mAb interfaces, to estimate alterations in bonding patterns between VEGFA and mAb because of their significant destabilizing effects on antigen-antibody interactions [57]. Furthermore, the variants were searched for their phenotypic effects as well as their reported association with any disease. The results obtained from each of these tools are based on statistical predictions and, consequently, are not supposed to be 100% accurate. Therefore, multiple tools as well as consensus decision making were used to minimize the likelihood of erroneous predictions. Despite some limitations regarding the accuracy of in silico methods compared to direct laboratory experimentation, these are quite useful to identify variants of greater significance and, therefore, can save resources, time, and efforts [58].
The binding affinity of BVZ for VEGFA was predicted to be affected by VEGFAR82Q, VEGFAG92R, as well as VEGFAR82W (Table 2). Both Arg82 and Gly92 were part of the functional epitope of VEGFA, however, the contribution of Gly92 to VEGFA-BVZ binding was around two folds greater than that of Arg82 [34]. VEGFAR82W variant caused structural changes, which resulted in a clash between VEGFA and BVZ, weakening their interaction (Table 4). This conformational change resulted in the loss of a H-bond between Trp82 (VEGFA) and Ser106 (BVZ Hchain) as well as the conversion of a cation-pi interaction into a H-bond interaction between Trp82 (VEGFA) andTyr102 (BVZ H chain) (Figures 3A and 3B). The cation-pi interaction is 2.5-5 times weaker than the H-bond interaction [55]. Therefore, these alterations may weaken the interaction between VEGFA and the BVZ H chain, which was in line with the findings of SAAMBE-3D and MutaBind2, which predicted a reduction in binding affinity. Although, no structural change was predicted for VEGFAR82Q variant, it caused the loss of contact between Gln88 of VEGFA and Gly104 of H chain of BVZ. Furthermore, three tools including PPcheck [45] predicted significant destabilizing effect on the binding affinity of BVZ H chain to VEGFA for the variant. The two flanking residues of Arg82 in VEGFA, Met81 and Ile83, are also part of the functional epitope of VEGFA, and alanine scanning analysis reported a significant decline in the binding affinity of BVZ for VEGFA with mutation at these two positions, indicating their sheer importance in the stabilization of the VEGFA-BVZ complex [34]. The destabilizing effect of both VEGFAR82Q and VEGFAR82W as well as the significance of the flanking residues of Arg82 in the binding affinity of BVZ for VEGFA implies that the residue is quite important for epitope-paratope interaction between VEGFA and BVZ.
VEGFAG92R substantially reduces the binding affinity of BVZ for the VEGFA, as predicted by all four tools used to analyze the alteration in binding affinities. This finding is well supported by the findings of the iCn3D structure viewer [46] and the Arpeggio Web Server [47]. This variant brought four residues (Pro100, Ser105, Ser106, and His107) of the BVZ H chain into contact with Arg92 of VEGFA and resulted in the loss of two H-bonds. Furthermore, this variant increased the buried surface area at the VEGFA-BVZ H chain interface significantly. BSA strongly correlates with the number of atoms exposed on the surface of antigen and antibody, as well as, to some extent, with the interaction enthalpy of protein-protein complexes and antigen-antibody complexes [59, 60]. In addition, significant alteration in buried surface area may serve as evidence for change in structure or conformation [32]. Therefore, it can be inferred that the VEGFAG92R variant significantly destabilizes the VEGFA-BVZ complex through conformational alteration. Only VEGFAR82Q caused an alteration in the antigen-antibody interface among the VEGFA variants for BVZ.
In this present study, among the five missense variants of VEGFA in the epitope for ranibizumab, only two (VEGFAR82W and VEGFAR82Q) were predicted to affect VEGFA and RBZ interactions, as indicated by reduced binding affinity (∆∆G > 1.0 kcal/mol) (Table 3). The VEGFAR82W variant was predicted to cause structural change in VEGFA, which brought Trp82 of VEGFAR82W and Thr105 of the RBZ H chain in close proximity (within 4 Å) unlike the wild type VEGFA (Figure 2A and 2B). The structural change resulting from this variant was also predicted to induce clashes between VEGFA and RBZ, further weakening the interaction (Table 4). In addition, this variant converted the cation-pi interaction between the amino acid residue at position 82 of VEGFA (Arg in wild-type and Trp in R82W) and Tyr102 of the RBZ H chain into a H-bond (Figures 4A and 4B). Because the H bond is 2.5 to 5 times weaker than the cation-pi interactions that stabilize antigen-antibody complexes, the change in bonding pattern could have contributed to the weakened interaction between VEGFA and RBZ [55, 61]. The R82Q variant may be considered to have lesser destabilizing effect on VEGFA-RBZ interaction than the R82W variant, as the loss of a cation-pi interaction present between the Arg82 of VEGFAWT and Tyr102 of the RBZ H chain was compensated to some extent by the formation of a new H-bond between the RBZ H chain and VEGFAR82Q, which was absent from VEGFAWT-RBZ H chain interaction (Figures 4A and 4C). This prediction was substantiated by the finding that the R82Q variant did not induce structural change unlike the R82W variant (Table 4). This variant also caused a loss of contact between Gln82 of VEGFAR82Q and Gly104 of the RBZ H chain. In addition, further evidence of the destabilizing effects of both R82W and R82Q variants came from the finding that these variants destroyed all the salt bridges between VEGFA and RBZ light chain (L chain) (Table 4). Furthermore, changes in the VEGFA and RBZ H chain interaction interface were observed for both of the variants.
The variants were analyzed for their deleterious phenotypic impact using four different tools. PolyPhen-2 utilizes both structure and sequence based predictive features, an alignment pipeline, and the ML method of classification to estimate the pathogenicity of missense variants [62]. SIFT (Sorting Intolerant From Tolerant) predicts effects of amino acid changes on protein function by relying only on sequence homology information [63]. The Meta-SNP algorithm combines four existing methods: PANTHER, PhD-SNP, SIFT as well as SNAP, and yields better performance than single predictor tools [48]. PredictSNP uses consensus prediction from six different tools (MAPP, PhD-SNP, PolyPhen-1, PolyPhen-2, SIFT and SNAP) and provides a relatively more accurate and robust prediction of the pathogenicity of variants [49]. Of the six missense variants, only VEGFAR82W was predicted by all four tools to be pathogenic. Therefore, it is highly likely that the R82W variant is associated with disease. However, no association of this variant with any disease has been reported. In addition, the VEGFAQ87R was found to be associated with fibrosarcoma [51]. Further studies are needed to elucidate the role of these variants in disease and associated conditions.
For contextualizing the findings of this study, the Pharmacogenomics Knowledge Base (PharmGKB) was searched to find BVZ and RBZ response related variants [64]. Thirty BVZ response-associated and eight RBZ response-associated unique SNPs with high significance were found in PharmGKB that were associated with either poor or better response. The evidence for all of these drug-variant associations comes from single significant studies, and the outcomes have not been replicated yet [64]. Six of the BVZ response-associated SNPs and four of the RBZ response-associated SNPs were found on the VEGFA gene. Therefore, an enormous gap exists in our knowledge about the role of anti-VEGFA mAb response-associated variants in determining therapeutic outcome. This study identified three epitopic missense variants of VEGFA which may be predictive of a poor therapeutic outcome of anti-VEGFA mAb treatment, irrespective of the disease being treated. Furthermore, this study reports the potential pathogenic effect of the rs114262569 variant for the first time. However, this study has certain limitations due to the use of multiple bioinformatic tools that provide binary predictions, for instance, beneficial or harmful, without explicit explanation about the causes or impacts of the change. In addition, analyses with bioinformatic tools cannot often predict the actual effect of variants in vivo as a multitude of factors play roles in determining the impact of a particular event that cannot be completely replicated in a computational system. Besides, for a few analyses, the 3D structures of VEGFA variants were predicted using homology-based modeling, which may not fully reflect the native, in vivo conformation of VEGFA. Nevertheless, this study identified certain variants among all existing polymorphisms of VEGFA that are likely to have significant destabilizing effects on VEGFA-mAb interaction and, therefore, may influence the clinical outcomes in patients treated with the mAbs. This study, therefore, can contribute as a roadmap for further research to delineate the detailed role of missense variants in patients’ response to BVZ and RBZ treatment.
CONCLUSION
High rate of non-responsiveness to therapeutic anti-VEGFA mAb (BVZ and RBZ), which are used to treat various cancers and ophthalmological conditions such as metastatic colorectal cancer, renal cell carcinoma, diabetic macular edema, was the inspiration behind this study. This in silico study identifies some candidate missense variants that may result in poor response to anti-VEGFA therapeutic mAb. Based on the findings of the present study, further research studies may be designed and carried out using in vitro and in vivo models in order to analyze the effects of these candidate missense variants on VEGFA-mAb interaction. Additionally, studies with patients currently undergoing anti-VEGFA mAb treatment for various diseases can be of help to shed more light on the underlying causes of heterogeneity of responsiveness. Through screening of these destabilizing variants in patients and selection of the more potent therapeutic mAb, whose action is not affected by the variant, for treatment, the clinical outcomes in patients can be improved.
ACKNOWLEDGEMENT
This study was conducted without a research grant.
AUTHOR CONTRIBUTIONS
Design of the work- SSS, TA, AAS; Acquisition, analysis, and interpretation of data- MTHT, SSS, KF, SUM; Manuscript preparation and reviewing- MTHT, SSS, AAS. All authors approved the final version of the manuscript.
References
- [1]Ferrara N. Vascular endothelial growth factor: basic science and clinical progress. Endocrine reviews. 2004;25:581-611.
- [2]Claesson-Welsh L, Welsh M. VEGFA and tumour angiogenesis. Journal of Internal Medicine. 2013;273:114-27.
- [3]Shibuya M. Vascular Endothelial Growth Factor (VEGF) and its receptor (VEGFR) signaling in angiogenesis: a crucial target for anti- and pro-angiogenic therapies. Genes & cancer. 2011;2:1097-105.
- [4]Zhou Y, Zhu X, Cui H, Shi J, Yuan G, Shi S, et al. The role of the VEGF family in coronary heart disease. Frontiers in cardiovascular medicine. 2021;8:738325.
- [5]Braile M, Marcella S, Cristinziano L, Galdiero MR, Modestino L, Ferrara AL, et al. VEGF-A in cardiomyocytes and heart diseases. International journal of molecular sciences. 2020;21:5294.
- [6]Neves KB, Rios FJ, van der Mey L, Alves-Lopes R, Cameron AC, Volpe M, et al. VEGFR (Vascular Endothelial Growth Factor Receptor) inhibition induces cardiovascular damage via redox-sensitive processes. Hypertension. 2018;71:638-47.
- [7]Goel HL, Mercurio AM. VEGF targets the tumour cell. Nature reviews Cancer. 2013;13:871-82.
- [8]Hanahan D, Weinberg RA. The hallmarks of cancer. Cell. 2000;100:57-70.
- [9]Matsumoto K, Ema M. Roles of VEGF-A signalling in development, regeneration, and tumours. Journal of biochemistry. 2014;156:1-10.
- [10]Ferrara N, Adamis AP. Ten years of anti-vascular endothelial growth factor therapy. Nature reviews Drug discovery. 2016;15:385-403.
- [12]Karaman S, Leppänen V-M, Alitalo K. Vascular endothelial growth factor signaling in development and disease. Development. 2018;145:dev151019.
- [13]Ferrara N, Gerber H-P, LeCouter J. The biology of VEGF and its receptors. Nature medicine. 2003;9:669- 76.
- [14]Lien S, Lowman HB. Therapeutic anti-VEGF antibodies. Handbook of experimental pharmacology. 2008:131-50
- [15]Garcia J, Hurwitz HI, Sandler AB, Miles D, Coleman RL, Deurloo R, et al. Bevacizumab (Avastin®) in cancer treatment: A review of 15 years of clinical experience and future outlook. Cancer treatment reviews. 2020;86:102017.
- [16]Ranieri G, Patruno R, Ruggieri E, Montemurro S, Valerio P, Ribatti D. Vascular endothelial growth factor (VEGF) as a target of bevacizumab in cancer: from the biology to the clinic. Current medicinal chemistry. 2006;13:1845-57.
- [17]Chellappan DK, Leng KL, Jia LJ, Aziz NABA, Hoong WC, Qian YC, et al. The role of bevacizumab on tumour angiogenesis and in the management of gynaecological cancers: A review. Biomedicine & pharmacotherapy. 2018;102:1127-44.
- [18]Lee A, Shirley M. Ranibizumab: A review in retinopathy of prematurity. Pediatric Drugs. 2021;23:111-7.
- [19]Ng DSC, Fung NSK, Yip FLT, Lai TYY. Ranibizumab for myopic choroidal neovascularization. Expert opinion on biological therapy. 2020;20:1385-93.
- [20]Blick SKA, Keating GM, Wagstaff AJ. Ranibizumab. Drugs. 2007;67:1199-9.
- [21]Kazazi-Hyseni F, Beijnen JH, Schellens JHM. Bevacizumab. The oncologist. 2010;15:819-25.
- [22]Chatziralli I. Ranibizumab for the treatment of diabetic retinopathy. Expert opinion on biological therapy. 2021;21:991-7.
- [23]Mullard A. FDA approves 100th monoclonal antibody product. Nature Reviews Drug Discovery. 2021;20:491-5.
- [24]Urup T, Gillberg L, Kaastrup K, Lü MJS, Michaelsen SR, Andrée LV, et al. Angiotensinogen promoter methylation predicts bevacizumab treatment response of patients with recurrent glioblastoma. Molecular Oncology. 2020;14:964-73.
- [25]Chuluunbat T, Chan RVP, Wang N-K, Lien R, Chen Y-P, Chao A-N, et al. Nonresponse and recurrence of retinopathy of prematurity after intravitreal Ranibizumab treatment. Ophthalmic Surgery, Lasers and Imaging Retina. 2016;47:1095-105.
- [26]Osathanugrah P, Sanjiv N, Siegel NH, Ness S, Chen X, Subramanian ML. The impact of race on shortterm treatment response to Bevacizumab in diabetic macular edema. American Journal of Ophthalmology. 2021;222:310-7.
- [27]Papachristos A, Karatza E, Kalofonos H, Sivolapenko G. Pharmacogenetics in model-based optimization of Bevacizumab therapy for metastatic colorectal cancer. International journal of molecular sciences. 2020;21:3753.
- [28]Chionh F, Gebski V, Al-Obaidi SJ, Mooi JK, Bruhn MA, Lee CK, et al. VEGF-A, VEGFR1 and VEGFR2 single nucleotide polymorphisms and outcomes from the AGITG MAX trial of capecitabine, bevacizumab and mitomycin C in metastatic colorectal cancer. Scientific reports. 2022;12:1238.
- [29]Cobos E, Recalde S, Anter J, Hernandez-Sanchez M, Barreales C, Olavarrieta L, et al. Association between CFH, CFB, ARMS2, SERPINF1, VEGFR1 and VEGF polymorphisms and anatomical and functional response to ranibizumab treatment in neovascular age-related macular degeneration. Acta ophthalmologica. 2018;96:e201-e12.
- [30]Zhao L, Grob S, Avery R, Kimura A, Pieramici D, Lee J, et al. Common variant in VEGFA and response to anti-VEGF therapy for neovascular age-related macular degeneration. Current molecular medicine. 2013;13:929-34.
- [31]Krispel C, Rodrigues M, Xin X, Sodhi A. Ranibizumab in diabetic macular edema. World journal of diabetes. 2013;4:310-8.
- [32]Ahsan T, Sajib AA. Missense variants in the TNFA epitopes and their effects on interaction with therapeutic antibodies—in silico analysis. Journal of Genetic Engineering and Biotechnology. 2022;20:7.
- [33]Raybould MIJ, Marks C, Lewis AP, Shi J, Bujotzek A, Taddese B, et al. Thera-SAbDab: the Therapeutic Structural Antibody Database. Nucleic acids research. 2020;48:D383-D8.
- [34]Muller YA, Chen Y, Christinger HW, Li B, Cunningham BC, Lowman HB, et al. VEGF and the Fab fragment of a humanized neutralizing antibody: crystal structure of the complex at 2.4 A resolution and mutational analysis of the interface. Structure. 1998;6:1153-67
- [35]Howe KL, Achuthan P, Allen J, Allen J, Alvarez-Jarreta J, Amode MR, et al. Ensembl 2021. Nucleic acids research. 2021;49:D884-D91.
- [36]Berman HM. The Protein Data Bank. Nucleic Acids Research. 2000;28:235-42.
- [37]Pettersen EF, Goddard TD, Huang CC, Couch GS, Greenblatt DM, Meng EC, et al. UCSF Chimera- a visualization system for exploratory research and analysis. Journal of computational chemistry. 2004;25:1605-12.
- [38]Ittisoponpisan S, Islam SA, Khanna T, Alhuzimi E, David A, Sternberg MJE. Can predicted protein 3D structures provide reliable insights into whether missense variants are disease associated? Journal of Molecular Biology. 2019;431:2197-212.
- [39]Rodrigues CHM, Myung Y, Pires DEV, Ascher DB. mCSM-PPI2: predicting the effects of mutations on protein–protein interactions. Nucleic Acids Research. 2019;47:W338-W44.
- [40]Pahari S, Li G, Murthy AK, Liang S, Fragoza R, Yu H, et al. SAAMBE-3D: predicting effect of mutations on protein-protein interactions. International journal of molecular sciences. 2020;21:2563.
- [41]Zhang N, Chen Y, Lu H, Zhao F, Alvarez RV, Goncearenco A, et al. MutaBind2: predicting the impacts of single and multiple mutations on protein-protein interactions. iScience. 2020;23:100939.
- [42]Dehouck Y, Kwasigroch JM, Rooman M, Gilis D. BeAtMuSiC: Prediction of changes in protein-protein binding affinity on mutations. Nucleic acids research. 2013;41:W333-9.
- [43]UniProt Consortium. UniProt: the universal protein knowledgebase in 2021. Nucleic Acids Research. 2021;49:D480-D9
- [44]Waterhouse A, Bertoni M, Bienert S, Studer G, Tauriello G, Gumienny R, et al. SWISS-MODEL: homology modelling of protein structures and complexes. Nucleic Acids Research. 2018;46:W296-W303.
- [45]Sukhwal A, Sowdhamini R. PPCheck: A webserver for the quantitative analysis of protein-protein interfaces and prediction of residue hotspots. Bioinformatics and biology insights. 2015;9:141-51.
- [46]Wang J, Youkharibache P, Zhang D, Lanczycki CJ, Geer RC, Madej T, et al. iCn3D, a web-based 3D viewer for sharing 1D/2D/3D representations of biomolecular structures. Bioinformatics. 2020;36:131-5.
- [47]Jubb HC, Higueruelo AP, Ochoa-Montaño B, Pitt WR, Ascher DB, Blundell TL. Arpeggio: A web server for calculating and visualising interatomic interactions in protein structures. Journal of Molecular Biology. 2017;429:365-71.
- [48]Capriotti E, Altman RB, Bromberg Y. Collective judgment predicts disease-associated single nucleotide variants. BMC genomics. 2013;14:S2.
- [49]Bendl J, Stourac J, Salanda O, Pavelka A, Wieben ED, Zendulka J, et al. PredictSNP: robust and accurate consensus classifier for prediction of disease-related mutations. PLoS computational biology. 2014;10:e1003440.
- [50]Kamat MA, Blackshaw JA, Young R, Surendran P, Burgess S, Danesh J, et al. PhenoScanner V2: an expanded tool for searching human genotype–phenotype associations. Bioinformatics. 2019;35:4851-3.
- [51]Piñero J, Bravo À, Queralt-Rosinach N, Gutiérrez-Sacristán A, Deu-Pons J, Centeno E, et al. DisGeNET: a comprehensive platform integrating information on human disease-associated genes and variants. Nucleic acids research. 2017;45:D833-D9
- [52]Brozzo MS, Bjelić S, Kisko K, Schleier T, Leppänen V-M, Alitalo K, et al. Thermodynamic and structural description of allosterically regulated VEGFR-2 dimerization. Blood. 2012;119:1781-8.
- [53]Brender JR, Zhang Y. Predicting the Effect of Mutations on Protein-Protein Binding Interactions through Structure-Based Interface Profiles. PLoS computational biology. 2015;11:e1004494.
- [54]Sirin S, Apgar JR, Bennett EM, Keating AE. AB-Bind: Antibody binding mutational database for computational affinity predictions. Protein science. 2016;25:393-409.
- [55]Xie N-Z, Du Q-S, Li J-X, Huang R-B. Exploring strong interactions in proteins with quantum chemistry and examples of their applications in drug design. PLOS ONE. 2015;10:e0137113.
- [56]Gyulkhandanyan A, Rezaie AR, Roumenina L, Lagarde N, Fremeaux-Bacchi V, Miteva MA, et al. Analysis of protein missense alterations by combining sequence- and structure-based methods. Molecular Genetics & Genomic Medicine. 2020;8:e1166.
- [57]Nguyen MN, Pradhan MR, Verma C, Zhong P. The interfacial character of antibody paratopes: analysis of antibody–antigen structures. Bioinformatics. 2017;33:2971-6.
- [58]Brea-Fernandez A, Ferro M, Fernandez-Rozadilla C, Blanco A, Fachal L, Santamarina M, et al. An Update of In Silico Tools for the Prediction of Pathogenesis in Missense Variants. Current Bioinformatics. 2011;6:185-98
- [59]Jackson RM. Comparison of protein-protein interactions in serine protease-inhibitor and antibodyantigen complexes: Implications for the protein docking problem. Protein Science. 2008;8:603-13.
- [60]Marillet S, Lefranc M-P, Boudinot P, Cazals F. Dissecting Interfaces of Antibody-Antigen Complexes: from Ligand Specific Features to Binding Affinity Predictions.
- [61]Dalkas GA, Teheux F, Kwasigroch JM, Rooman M. Cation–π, amino–π, π–π, and H‐bond interactions stabilize antigen–antibody interfaces. Proteins: Structure, Function, and Bioinformatics. 2014;82:1734-46.
- [62]Adzhubei IA, Schmidt S, Peshkin L, Ramensky VE, A. G, P. B, et al. A method and server for predicting damaging missense mutations. Nature methods. 2010;7:248-9.
- [63]Ng PC, Henikoff S. SIFT: Predicting amino acid changes that affect protein function. Nucleic acids research. 2003;31:3812-4.
- [64]Whirl-Carrillo M, Huddart R, Gong L, Sangkuhl K, Thorn CF, Whaley R, et al. An evidence-based framework for evaluating Pharmacogenomics Knowledge for personalized medicine. Clinical pharmacology and therapeutics. 2021;110:563-72.